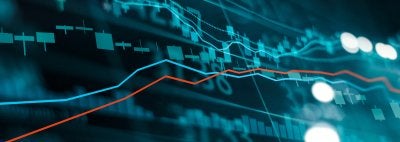
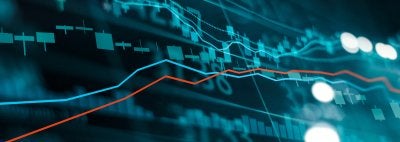
Mathematical modeling during the COVID-19 pandemic, QnA with COE's Gregory Hancock
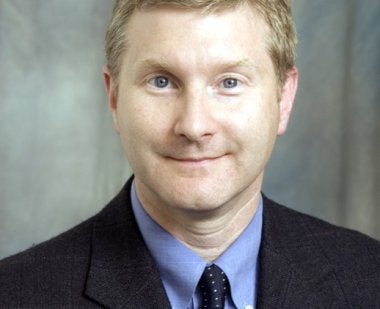
Mathematical modeling is a useful tool in predicting patterns and approximating reality. Based on assumptions and data, modeling can provide valuable insight into world events, such as the COVID-19 pandemic, where models have informed policy and public health strategies. In context of the pandemic, Dr. Gregory R. Hancock, director of the Measurement, Statistics & Evaluation program at COE, discusses the foundation for modeling, how projections are evaluated, and why modeling is an effective tool, even when it misses the mark.
Which types of information and data are used in modeling, and how does this play out in modeling for Covid-19?
Models are typically built based on a mathematical understanding of the general nature of a phenomenon—exponential growth for viruses, for instance. Additionally, assumptions, such as whether COVID-19 transmission is or is not like SARS and whether COVID-19 is seasonal or not, as well as the role of environmental changes, such as closing schools and stay-at-home orders, contribute to constructing models. Models are constantly updated as new data come in, and when models differ in any combination of underlying assumptions, as current models do, the projections can be wildly different.
How does real-time modeling present unique challenges?
Real-time modeling can be done very effectively when you have reliable sources of data, an understanding of how incoming data will behave over time, and when environments have experience reacting to the phenomenon. In the current pandemic, we have many data sources that are currently unreliable and nonrepresentative, a very weak understanding of how data will behave due to, as-yet, a limited understanding of transmissibility and fatality, and constantly-changing and untested health policies and messaging to which people differ in their subsequent behavioral choices.
How should people interpret or evaluate projections from models?
With regard to current COVID-19 models, the data upon which they are based are still too unreliable, the relevant variables and their interplay are still not well-understood, and the environment is still too dynamic. For these reasons, despite epidemiologists’ and economists’ best efforts and intentions, current models are yielding wildly different predictions, and we do not yet have any idea which, if any, should be regarded as accurate.
Can you describe a specific example of how a recent model influenced community, behavior, policy or other changes related to the pandemic?
All of the decisions we are making currently are informed by models. Closing schools, social distancing, stay-at-home orders, invoking the Defense Production Act to make ventilators – all of these decisions are based on models by the CDC, WHO, and numerous other national and international agencies, models that describe the spread of COVID-19 as well as the expected numbers of hospitalizations.
Why are models helpful, even though they might be wrong?
There is a famous quote by statistician George Box: “All models are wrong, but some are useful.” To elaborate briefly, most systems simply have too many moving, interacting parts to get it exactly right. But the model might still make useful predictions to help plan. For example, a model of airborne virus transmission derived from a previous outbreak, even though not identical in time and place, can definitely help to prepare for what might lay ahead under current circumstances.