INFORMATIONAL VIDEOS:
- QMMS Program Structure
- QMMS Program Culture
- QMMS Student Placement and Achievement
- Message from QMMS Graduate Students
FAST FACTS:
- Our program has a new name! Quantitative Methodology: Measurement and Statistics (QMMS) replaces the previous program name and abbreviation -- Measurement, Statistics and Evaluation (EDMS).
- Funded students get valuable experience as departmental research or teaching assistants, as graduate assistants in affiliated areas on campus, or on contract/assistantship work in partnership with research/testing agencies in the Washington, DC area.
- Graduate students are heavily involved in research projects, with over 80% presenting at national and international conferences, and many publishing in leading academic journals. Within the last five years alone our students have had first-authored methodological papers appear in, or accepted for publication in, such journals as Multivariate Behavioral Research, Psychological Methods, Journal of Educational Measurement, Journal of Education and Behavioral Statistics, Applied Psychological Measurement, International Journal of Testing, Educational and Psychological Measurement, Frontiers in Psychology (Quantitative Psychology and Measurement section), and Journal of Experimental Education.
- Over half of our Ph.D. students get summer internships (e.g., College Board, Educational Testing Service, American College Testing, Measured Progress) and/or dissertation fellowships (American Educational Research Association, Spencer Foundation, Society of Multivariate Experimental Psychology, Educational Testing Service).
- Graduates of our program get academic positions all over the country (e.g., Arizona State University, University of Georgia, University of Texas, University of Minnesota, Georgetown University, The George Washington University, University of North Carolina, University of Hawaii, University of Denver), as well as jobs at leading research agencies (e.g., Educational Testing Service, American College Testing, Westat, American Institutes for Research, Stanford Research International, Mathematica, Association of American Medical Colleges, Google, Duolingo).
- Our faculty include award-winning mentors, Fellows in leading national associations, and editors/editorial board members on such leading journals as Journal of Educational Measurement, Journal of Educational and Behavioral Statistics, Structural Equation Modeling: A Multidisciplinary Journal, Multivariate Behavioral Research, Psychometrika, Psychological Methods, Journal of Research on Educational Effectiveness, and AERA Open.
![]() | Gregory R. Hancock 1230D Benjamin Bldg. | ||||||||||||||||||||||||||||||||||||||||||||||||||||||||||||||||||||||||||||||||||||||||||||||||||||||||||||||||||||||||||||||||||||||||||||||||||||||||||||||||||||||||||||||||||||||||||||||||||||||||||||||||||||||||||||||||||||||||||||||||||||||||||||||||||||||||||||||||||||||||||||||||||||||||||||||||||||||||||||||||||||||||||||||||||||||||||||||||||||||||||||||||||||||||||||||||||||||||||||||||||||||||||||||||||||||||||||||||||||||||||||||||||||||||||||||||||||||||||||||||||||||||||||||||||||||||||||||||||||||||||||||||||||||||||||||||||||||||||||||||||||||||||||||||||||||||||||||||||||||||||||||||||||||||||||||||||||||||||||||||||||||||||||||||||||||||||||||||||||||||||||||||||||||||||||||||||||||||||||||||||||||||||||||||||||||||||||||||||||||||||||||||||||||||||||||||||||||||||||||||||||||||||||||||||||||||||||||||||||||||||||||||||||||||||||||||||||||||||||||||||||||||||||||||||||||||||||||||||||||||||||||||||||||||||||||||||||||||||||||||||||||||||||||||||||||||||||||||||||||
![]() | Jeffrey Harring 1230E Benjamin Building | ||||||||||||||||||||||||||||||||||||||||||||||||||||||||||||||||||||||||||||||||||||||||||||||||||||||||||||||||||||||||||||||||||||||||||||||||||||||||||||||||||||||||||||||||||||||||||||||||||||||||||||||||||||||||||||||||||||||||||||||||||||||||||||||||||||||||||||||||||||||||||||||||||||||||||||||||||||||||||||||||||||||||||||||||||||||||||||||||||||||||||||||||||||||||||||||||||||||||||||||||||||||||||||||||||||||||||||||||||||||||||||||||||||||||||||||||||||||||||||||||||||||||||||||||||||||||||||||||||||||||||||||||||||||||||||||||||||||||||||||||||||||||||||||||||||||||||||||||||||||||||||||||||||||||||||||||||||||||||||||||||||||||||||||||||||||||||||||||||||||||||||||||||||||||||||||||||||||||||||||||||||||||||||||||||||||||||||||||||||||||||||||||||||||||||||||||||||||||||||||||||||||||||||||||||||||||||||||||||||||||||||||||||||||||||||||||||||||||||||||||||||||||||||||||||||||||||||||||||||||||||||||||||||||||||||||||||||||||||||||||||||||||||||||||||||||||||||||||||||||
![]() | Hong Jiao 1230C Benjamin Building | ||||||||||||||||||||||||||||||||||||||||||||||||||||||||||||||||||||||||||||||||||||||||||||||||||||||||||||||||||||||||||||||||||||||||||||||||||||||||||||||||||||||||||||||||||||||||||||||||||||||||||||||||||||||||||||||||||||||||||||||||||||||||||||||||||||||||||||||||||||||||||||||||||||||||||||||||||||||||||||||||||||||||||||||||||||||||||||||||||||||||||||||||||||||||||||||||||||||||||||||||||||||||||||||||||||||||||||||||||||||||||||||||||||||||||||||||||||||||||||||||||||||||||||||||||||||||||||||||||||||||||||||||||||||||||||||||||||||||||||||||||||||||||||||||||||||||||||||||||||||||||||||||||||||||||||||||||||||||||||||||||||||||||||||||||||||||||||||||||||||||||||||||||||||||||||||||||||||||||||||||||||||||||||||||||||||||||||||||||||||||||||||||||||||||||||||||||||||||||||||||||||||||||||||||||||||||||||||||||||||||||||||||||||||||||||||||||||||||||||||||||||||||||||||||||||||||||||||||||||||||||||||||||||||||||||||||||||||||||||||||||||||||||||||||||||||||||||||||||||||
![]() | Yang Liu 1230B Benjamin Building | ||||||||||||||||||||||||||||||||||||||||||||||||||||||||||||||||||||||||||||||||||||||||||||||||||||||||||||||||||||||||||||||||||||||||||||||||||||||||||||||||||||||||||||||||||||||||||||||||||||||||||||||||||||||||||||||||||||||||||||||||||||||||||||||||||||||||||||||||||||||||||||||||||||||||||||||||||||||||||||||||||||||||||||||||||||||||||||||||||||||||||||||||||||||||||||||||||||||||||||||||||||||||||||||||||||||||||||||||||||||||||||||||||||||||||||||||||||||||||||||||||||||||||||||||||||||||||||||||||||||||||||||||||||||||||||||||||||||||||||||||||||||||||||||||||||||||||||||||||||||||||||||||||||||||||||||||||||||||||||||||||||||||||||||||||||||||||||||||||||||||||||||||||||||||||||||||||||||||||||||||||||||||||||||||||||||||||||||||||||||||||||||||||||||||||||||||||||||||||||||||||||||||||||||||||||||||||||||||||||||||||||||||||||||||||||||||||||||||||||||||||||||||||||||||||||||||||||||||||||||||||||||||||||||||||||||||||||||||||||||||||||||||||||||||||||||||||||||||||||||
![]() | Laura Stapleton 3304 Benjamin Building | ||||||||||||||||||||||||||||||||||||||||||||||||||||||||||||||||||||||||||||||||||||||||||||||||||||||||||||||||||||||||||||||||||||||||||||||||||||||||||||||||||||||||||||||||||||||||||||||||||||||||||||||||||||||||||||||||||||||||||||||||||||||||||||||||||||||||||||||||||||||||||||||||||||||||||||||||||||||||||||||||||||||||||||||||||||||||||||||||||||||||||||||||||||||||||||||||||||||||||||||||||||||||||||||||||||||||||||||||||||||||||||||||||||||||||||||||||||||||||||||||||||||||||||||||||||||||||||||||||||||||||||||||||||||||||||||||||||||||||||||||||||||||||||||||||||||||||||||||||||||||||||||||||||||||||||||||||||||||||||||||||||||||||||||||||||||||||||||||||||||||||||||||||||||||||||||||||||||||||||||||||||||||||||||||||||||||||||||||||||||||||||||||||||||||||||||||||||||||||||||||||||||||||||||||||||||||||||||||||||||||||||||||||||||||||||||||||||||||||||||||||||||||||||||||||||||||||||||||||||||||||||||||||||||||||||||||||||||||||||||||||||||||||||||||||||||||||||||||||||||
Peter Steiner 1233 Benjamin Building | |||||||||||||||||||||||||||||||||||||||||||||||||||||||||||||||||||||||||||||||||||||||||||||||||||||||||||||||||||||||||||||||||||||||||||||||||||||||||||||||||||||||||||||||||||||||||||||||||||||||||||||||||||||||||||||||||||||||||||||||||||||||||||||||||||||||||||||||||||||||||||||||||||||||||||||||||||||||||||||||||||||||||||||||||||||||||||||||||||||||||||||||||||||||||||||||||||||||||||||||||||||||||||||||||||||||||||||||||||||||||||||||||||||||||||||||||||||||||||||||||||||||||||||||||||||||||||||||||||||||||||||||||||||||||||||||||||||||||||||||||||||||||||||||||||||||||||||||||||||||||||||||||||||||||||||||||||||||||||||||||||||||||||||||||||||||||||||||||||||||||||||||||||||||||||||||||||||||||||||||||||||||||||||||||||||||||||||||||||||||||||||||||||||||||||||||||||||||||||||||||||||||||||||||||||||||||||||||||||||||||||||||||||||||||||||||||||||||||||||||||||||||||||||||||||||||||||||||||||||||||||||||||||||||||||||||||||||||||||||||||||||||||||||||||||||||||||||||||||||||
![]() | Tracy Sweet 1229 Benjamin Building | ||||||||||||||||||||||||||||||||||||||||||||||||||||||||||||||||||||||||||||||||||||||||||||||||||||||||||||||||||||||||||||||||||||||||||||||||||||||||||||||||||||||||||||||||||||||||||||||||||||||||||||||||||||||||||||||||||||||||||||||||||||||||||||||||||||||||||||||||||||||||||||||||||||||||||||||||||||||||||||||||||||||||||||||||||||||||||||||||||||||||||||||||||||||||||||||||||||||||||||||||||||||||||||||||||||||||||||||||||||||||||||||||||||||||||||||||||||||||||||||||||||||||||||||||||||||||||||||||||||||||||||||||||||||||||||||||||||||||||||||||||||||||||||||||||||||||||||||||||||||||||||||||||||||||||||||||||||||||||||||||||||||||||||||||||||||||||||||||||||||||||||||||||||||||||||||||||||||||||||||||||||||||||||||||||||||||||||||||||||||||||||||||||||||||||||||||||||||||||||||||||||||||||||||||||||||||||||||||||||||||||||||||||||||||||||||||||||||||||||||||||||||||||||||||||||||||||||||||||||||||||||||||||||||||||||||||||||||||||||||||||||||||||||||||||||||||||||||||||||||
![]() | Ji Seung Yang 1225 Benjamin Building | ||||||||||||||||||||||||||||||||||||||||||||||||||||||||||||||||||||||||||||||||||||||||||||||||||||||||||||||||||||||||||||||||||||||||||||||||||||||||||||||||||||||||||||||||||||||||||||||||||||||||||||||||||||||||||||||||||||||||||||||||||||||||||||||||||||||||||||||||||||||||||||||||||||||||||||||||||||||||||||||||||||||||||||||||||||||||||||||||||||||||||||||||||||||||||||||||||||||||||||||||||||||||||||||||||||||||||||||||||||||||||||||||||||||||||||||||||||||||||||||||||||||||||||||||||||||||||||||||||||||||||||||||||||||||||||||||||||||||||||||||||||||||||||||||||||||||||||||||||||||||||||||||||||||||||||||||||||||||||||||||||||||||||||||||||||||||||||||||||||||||||||||||||||||||||||||||||||||||||||||||||||||||||||||||||||||||||||||||||||||||||||||||||||||||||||||||||||||||||||||||||||||||||||||||||||||||||||||||||||||||||||||||||||||||||||||||||||||||||||||||||||||||||||||||||||||||||||||||||||||||||||||||||||||||||||||||||||||||||||||||||||||||||||||||||||||||||||||||||||||
EMERITUS, ADJUNCT, and AFFILIATE FACULTY | |||||||||||||||||||||||||||||||||||||||||||||||||||||||||||||||||||||||||||||||||||||||||||||||||||||||||||||||||||||||||||||||||||||||||||||||||||||||||||||||||||||||||||||||||||||||||||||||||||||||||||||||||||||||||||||||||||||||||||||||||||||||||||||||||||||||||||||||||||||||||||||||||||||||||||||||||||||||||||||||||||||||||||||||||||||||||||||||||||||||||||||||||||||||||||||||||||||||||||||||||||||||||||||||||||||||||||||||||||||||||||||||||||||||||||||||||||||||||||||||||||||||||||||||||||||||||||||||||||||||||||||||||||||||||||||||||||||||||||||||||||||||||||||||||||||||||||||||||||||||||||||||||||||||||||||||||||||||||||||||||||||||||||||||||||||||||||||||||||||||||||||||||||||||||||||||||||||||||||||||||||||||||||||||||||||||||||||||||||||||||||||||||||||||||||||||||||||||||||||||||||||||||||||||||||||||||||||||||||||||||||||||||||||||||||||||||||||||||||||||||||||||||||||||||||||||||||||||||||||||||||||||||||||||||||||||||||||||||||||||||||||||||||||||||||||||||||||||||||||||
![]() | C. Mitchell Dayton | ||||||||||||||||||||||||||||||||||||||||||||||||||||||||||||||||||||||||||||||||||||||||||||||||||||||||||||||||||||||||||||||||||||||||||||||||||||||||||||||||||||||||||||||||||||||||||||||||||||||||||||||||||||||||||||||||||||||||||||||||||||||||||||||||||||||||||||||||||||||||||||||||||||||||||||||||||||||||||||||||||||||||||||||||||||||||||||||||||||||||||||||||||||||||||||||||||||||||||||||||||||||||||||||||||||||||||||||||||||||||||||||||||||||||||||||||||||||||||||||||||||||||||||||||||||||||||||||||||||||||||||||||||||||||||||||||||||||||||||||||||||||||||||||||||||||||||||||||||||||||||||||||||||||||||||||||||||||||||||||||||||||||||||||||||||||||||||||||||||||||||||||||||||||||||||||||||||||||||||||||||||||||||||||||||||||||||||||||||||||||||||||||||||||||||||||||||||||||||||||||||||||||||||||||||||||||||||||||||||||||||||||||||||||||||||||||||||||||||||||||||||||||||||||||||||||||||||||||||||||||||||||||||||||||||||||||||||||||||||||||||||||||||||||||||||||||||||||||||||||
![]() | Robert W. Lissitz | ||||||||||||||||||||||||||||||||||||||||||||||||||||||||||||||||||||||||||||||||||||||||||||||||||||||||||||||||||||||||||||||||||||||||||||||||||||||||||||||||||||||||||||||||||||||||||||||||||||||||||||||||||||||||||||||||||||||||||||||||||||||||||||||||||||||||||||||||||||||||||||||||||||||||||||||||||||||||||||||||||||||||||||||||||||||||||||||||||||||||||||||||||||||||||||||||||||||||||||||||||||||||||||||||||||||||||||||||||||||||||||||||||||||||||||||||||||||||||||||||||||||||||||||||||||||||||||||||||||||||||||||||||||||||||||||||||||||||||||||||||||||||||||||||||||||||||||||||||||||||||||||||||||||||||||||||||||||||||||||||||||||||||||||||||||||||||||||||||||||||||||||||||||||||||||||||||||||||||||||||||||||||||||||||||||||||||||||||||||||||||||||||||||||||||||||||||||||||||||||||||||||||||||||||||||||||||||||||||||||||||||||||||||||||||||||||||||||||||||||||||||||||||||||||||||||||||||||||||||||||||||||||||||||||||||||||||||||||||||||||||||||||||||||||||||||||||||||||||||||
![]() | George Macready | ||||||||||||||||||||||||||||||||||||||||||||||||||||||||||||||||||||||||||||||||||||||||||||||||||||||||||||||||||||||||||||||||||||||||||||||||||||||||||||||||||||||||||||||||||||||||||||||||||||||||||||||||||||||||||||||||||||||||||||||||||||||||||||||||||||||||||||||||||||||||||||||||||||||||||||||||||||||||||||||||||||||||||||||||||||||||||||||||||||||||||||||||||||||||||||||||||||||||||||||||||||||||||||||||||||||||||||||||||||||||||||||||||||||||||||||||||||||||||||||||||||||||||||||||||||||||||||||||||||||||||||||||||||||||||||||||||||||||||||||||||||||||||||||||||||||||||||||||||||||||||||||||||||||||||||||||||||||||||||||||||||||||||||||||||||||||||||||||||||||||||||||||||||||||||||||||||||||||||||||||||||||||||||||||||||||||||||||||||||||||||||||||||||||||||||||||||||||||||||||||||||||||||||||||||||||||||||||||||||||||||||||||||||||||||||||||||||||||||||||||||||||||||||||||||||||||||||||||||||||||||||||||||||||||||||||||||||||||||||||||||||||||||||||||||||||||||||||||||||||
![]() | Robert Mislevy | ||||||||||||||||||||||||||||||||||||||||||||||||||||||||||||||||||||||||||||||||||||||||||||||||||||||||||||||||||||||||||||||||||||||||||||||||||||||||||||||||||||||||||||||||||||||||||||||||||||||||||||||||||||||||||||||||||||||||||||||||||||||||||||||||||||||||||||||||||||||||||||||||||||||||||||||||||||||||||||||||||||||||||||||||||||||||||||||||||||||||||||||||||||||||||||||||||||||||||||||||||||||||||||||||||||||||||||||||||||||||||||||||||||||||||||||||||||||||||||||||||||||||||||||||||||||||||||||||||||||||||||||||||||||||||||||||||||||||||||||||||||||||||||||||||||||||||||||||||||||||||||||||||||||||||||||||||||||||||||||||||||||||||||||||||||||||||||||||||||||||||||||||||||||||||||||||||||||||||||||||||||||||||||||||||||||||||||||||||||||||||||||||||||||||||||||||||||||||||||||||||||||||||||||||||||||||||||||||||||||||||||||||||||||||||||||||||||||||||||||||||||||||||||||||||||||||||||||||||||||||||||||||||||||||||||||||||||||||||||||||||||||||||||||||||||||||||||||||||||||
![]() | William Schafer | ||||||||||||||||||||||||||||||||||||||||||||||||||||||||||||||||||||||||||||||||||||||||||||||||||||||||||||||||||||||||||||||||||||||||||||||||||||||||||||||||||||||||||||||||||||||||||||||||||||||||||||||||||||||||||||||||||||||||||||||||||||||||||||||||||||||||||||||||||||||||||||||||||||||||||||||||||||||||||||||||||||||||||||||||||||||||||||||||||||||||||||||||||||||||||||||||||||||||||||||||||||||||||||||||||||||||||||||||||||||||||||||||||||||||||||||||||||||||||||||||||||||||||||||||||||||||||||||||||||||||||||||||||||||||||||||||||||||||||||||||||||||||||||||||||||||||||||||||||||||||||||||||||||||||||||||||||||||||||||||||||||||||||||||||||||||||||||||||||||||||||||||||||||||||||||||||||||||||||||||||||||||||||||||||||||||||||||||||||||||||||||||||||||||||||||||||||||||||||||||||||||||||||||||||||||||||||||||||||||||||||||||||||||||||||||||||||||||||||||||||||||||||||||||||||||||||||||||||||||||||||||||||||||||||||||||||||||||||||||||||||||||||||||||||||||||||||||||||||||||
Kathryn Alvestad
| |||||||||||||||||||||||||||||||||||||||||||||||||||||||||||||||||||||||||||||||||||||||||||||||||||||||||||||||||||||||||||||||||||||||||||||||||||||||||||||||||||||||||||||||||||||||||||||||||||||||||||||||||||||||||||||||||||||||||||||||||||||||||||||||||||||||||||||||||||||||||||||||||||||||||||||||||||||||||||||||||||||||||||||||||||||||||||||||||||||||||||||||||||||||||||||||||||||||||||||||||||||||||||||||||||||||||||||||||||||||||||||||||||||||||||||||||||||||||||||||||||||||||||||||||||||||||||||||||||||||||||||||||||||||||||||||||||||||||||||||||||||||||||||||||||||||||||||||||||||||||||||||||||||||||||||||||||||||||||||||||||||||||||||||||||||||||||||||||||||||||||||||||||||||||||||||||||||||||||||||||||||||||||||||||||||||||||||||||||||||||||||||||||||||||||||||||||||||||||||||||||||||||||||||||||||||||||||||||||||||||||||||||||||||||||||||||||||||||||||||||||||||||||||||||||||||||||||||||||||||||||||||||||||||||||||||||||||||||||||||||||||||||||||||||||||||||||||||||||||||
![]() | Andre Rupp | ||||||||||||||||||||||||||||||||||||||||||||||||||||||||||||||||||||||||||||||||||||||||||||||||||||||||||||||||||||||||||||||||||||||||||||||||||||||||||||||||||||||||||||||||||||||||||||||||||||||||||||||||||||||||||||||||||||||||||||||||||||||||||||||||||||||||||||||||||||||||||||||||||||||||||||||||||||||||||||||||||||||||||||||||||||||||||||||||||||||||||||||||||||||||||||||||||||||||||||||||||||||||||||||||||||||||||||||||||||||||||||||||||||||||||||||||||||||||||||||||||||||||||||||||||||||||||||||||||||||||||||||||||||||||||||||||||||||||||||||||||||||||||||||||||||||||||||||||||||||||||||||||||||||||||||||||||||||||||||||||||||||||||||||||||||||||||||||||||||||||||||||||||||||||||||||||||||||||||||||||||||||||||||||||||||||||||||||||||||||||||||||||||||||||||||||||||||||||||||||||||||||||||||||||||||||||||||||||||||||||||||||||||||||||||||||||||||||||||||||||||||||||||||||||||||||||||||||||||||||||||||||||||||||||||||||||||||||||||||||||||||||||||||||||||||||||||||||||||||||
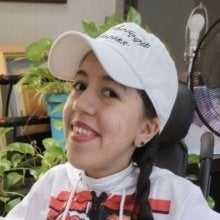
Marieh Arnett
M.S. student
marnett1@umd.edu
I received a B.S. in Psychology with a minor in Spanish and a B.S. in Statistics in 2019 from University of Maryland, College Park. I stayed at UMD and graduated with my Master of Professional Science in Clinical Psychological Science in 2020. My research interests from my psychology background are the effects that psychosis and psychotic symptoms/disorders have on interpersonal relationships, and my research interests from my statistics background are causal inference and mediation/moderation analyses. I’m super excited to be a part of the QMMS program!

Victor Eyo Essien
Ph.D. student
vessien@umd.edu
I have led monitoring and evaluation research for developmental projects for national and international non-governmental organizations in Nigeria. Also, I was involved in assessing students as an assistant examiner for the West African Examination Council (WAEC) and National Examination Council (NECO) for over a decade. My research interest lies in Educational Assessment, Item Response Theory, Item Development and Validation.
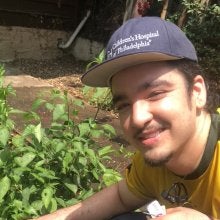
Charlie Fisk
Ph.D. student
fisk@umd.edu
B.S. in physics & mathematics, Penn State. I started my research career in the Children's Hospital of Philadelphia's radiology lab studying auditory steady-state responses in children with autism and adults with schizophrenia. I returned to school to study research methodology, graduating from Vanderbilt's quantitative methods master's program in 2019. Along the way I forged connections with the Vanderbilt School of Medicine where I worked on scale development and latent growth modeling. I am very excited to be here in the QMMS program where I can work and grow as a research methodologist.
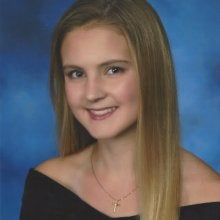
Victoria Haass
M.S. student
vhaass@umd.edu
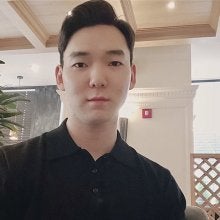
Youngjin Han
Ph.D. student
younghan@umd.edu
I am a Ph.D. student in the QMMS program with research interests in latent variable models focusing on item response theory, measurement error, and computerized adaptive testing. Before joining the program, I received a master's degree in Education and a bachelor's degree double majoring in Education and Applied Statistics both from Yonsei University, South Korea.
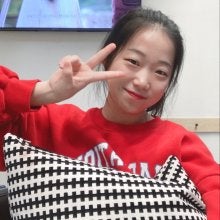
Youmin Hong
Ph.D. student
ymhong@umd.edu
I am a Ph.D. student in the QMMS program. I graduated with a Bachelor's degree in education and a Master's degree in education from Yonsei University, South Korea. My research interests lie in educational measurement and statistics focusing on the IRT model and its advances.

Ashani Setha Jayasekera
Ph.D. student
ajayase1@umd.edu
I graduated from the University of Maryland, Baltimore County in 2020 with a bachelor’s degree in Mathematics and minors in Statistics and Psychology. While there I worked in the Pediatric Psychology Lab researching the impact of COVID-19 on undergraduate students' learning during the initial shutdown in the spring of 2020. My experiences with data and answering research questions using different analytic methods to answer led me to the QMMS program and pursuing my master’s degree in quantitative methodology, and now my Ph.D.
Tessa Johnson
Ph.D. student
johnsont@umd.edu
I am a PhD student in the QMMS program with a methods background in latent variable models and an applied background in prevention science. I got my bachelor's degree in psychology from the University of Georgia in Athens and my master's in counseling and educational research from Georgia State University. My statistical research is focused on the development of methodologies used in the social, behavioral, and health sciences, especially in the areas of longitudinal processes and unobserved population heterogeneity.
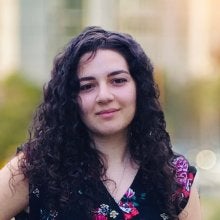
Margret Katsakhyan
M.S. student
margretk@umd.edu
I have a BS in Mathematics Education, and an MA in International Education Policy from UMD. After spending many years in instruction and advising from secondary to higher education my interest in quantitative research has led me to the QMMS program.
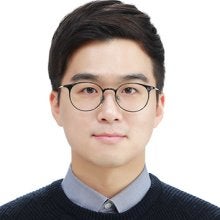
Muwon Kwon
Ph.D. student
mwkwon21@umd.edu
I am a Ph.D. student in the QMMS program. My research interest is causal inference focusing on research designs and machine learning using causal graphs. I obtained a Bachelor's degree double majoring in Education and Applied Statistics and a Master's degree in the Quantitative Method program in the Education department both from Yonsei University, South Korea.
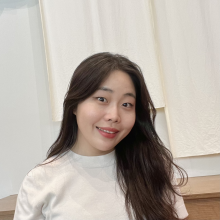
Dayeon Lee
Ph.D. student
daylee@umd.edu
I obtained my Master's degree in Educational Measurement & Evaluation from Seoul National University and am now working toward my Ph.D. in the QMMS program. Before starting my academic studies in the U.S., I was an elementary school teacher in South Korea. That hands-on teaching experience has greatly influenced my research interests. I'm keen on developing and extending quantitative methods to analyze various data, with the goal of better understanding real-world dynamics.

Hyeonseong Lee
Ph.D. student
hslee24@umd.edu
Before starting the QMMS Ph.D. program, I received both a Bachelor’s and Master’s degree in Education from Ewha Womans University, where I specialized in quantitative research methods. My academic interests center around measurement, with a particular focus on Item Response Theory and the analysis of latent variables.

Jung-Jung Lee
Ph.D. student
jlee1256@umd.edu
I received both my Bachelor’s in psychology and my Master’s in Industrial and Organizational Psychology from California State University, San Bernardino in 2014 and 2016. After I obtained my Master’s degree, I worked as a Workforce Research Analyst with the primary duty of reporting labor market data for the local Southern California region surrounding a community college. My strong interest in Item Response Theory has pushed me to further my education to the next level and I joined QMMS as a doctoral student in 2017.
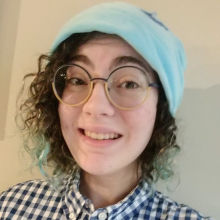
Quentin Leifer (pronouns: per/per/pers)
M.S. student
leifer5@umd.edu
I graduated from Stony Brook University in 2020 with a B.A. in psychology with concentrations in mathematics and philosophy. During my undergraduate career, I gained experience as a research assistant in Dr. Zelinsky’s Eye Lab and Dr. Luhmann’s Computational Behavior Lab. My current interests include psychological assessment, psychometrics, and disability research. As a disability student myself, I hope to give back to the community by assessing/conducting beneficial and necessary research.
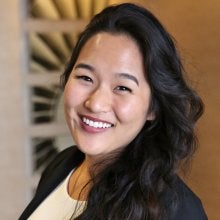
Pearl Lo
M.S. student
pearllo@umd.edu
I earned my bachelor's degree in Communication and Cinema & Media Studies from the University of Pennsylvania, where I also earned my master’s in Higher Education. I am currently a doctoral candidate in the Higher Education, Student Affairs, & International Education Policy Program at UMD. I was initially pursuing a certificate in the QMMS program, but enjoyed my QMMS classes so much that I ended up applying to the master’s program and am now thrilled to be a dual-degree student. I also work full-time at UMD as the Assistant Director for Assessment and Research for the Stamp Student Union. My research interests include cross-racial interactions and interracial friendship groups on college campuses, college admissions, and quantitative criticalism.

Elyssa Kristine Marie Granados Lou
M.S. student
elou1@umd.edu
I graduated from the University of Maryland, Baltimore County in 2022 with a Bachelor’s degree in Psychology. During that time, I worked in the Culture, Child and Adolescent Development (CCAD) Lab, Innovating Self-Perception, -Introspection, -Externalization (I-SPIE) Lab, and the Making Words Count Lab. I completed my honors thesis on factors that encourage and inhibit mental health help-seeking behaviors among Filipino Americans. Additionally, I have experience as a Registered Behavior Technician and a substitute teacher for Montgomery County Public School. I am now pursuing a Master’s degree in QMMS and am in my second year. For the 2024-2025 academic year, I am serving as the Co-Chair of Programming for the HDQM Graduate Student Association (GSA).
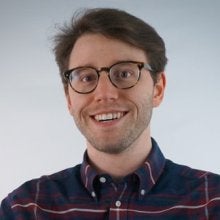
Christian Meyer
Ph.D. student
meyerct@umd.edu
Before starting the QMMS doctoral program, I studied physics at Creighton University in Omaha, Nebraska. After graduating, I received an Intramural Research Training Award (IRTA) from the National Institute of Mental Health (NIMH) to obtain additional research and methodological training. I then worked as a faculty research assistant in the Department of Psychology at UMD researching the neurological underpinnings of cognitive and emotional processes. Through these experiences, I developed an interest in understanding the theoretical basis for statistical methodology.

Sydney Peters
M.S. student
sjpeters@umd.edu
I am a first year master's student in the QMMS program. Previously, I earned a B.S. in Psychology with a minor in Statistics from Penn State University. My current research interests include psychological and educational measurement as well as how AI can be utilized to improve assessments.
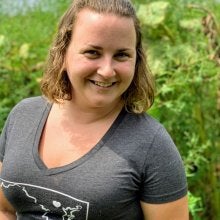
Mary Petras
M.S. student
mpetras@umd.edu
Prior to joining the QMMS community at UMD, I attended Duquesne University in Pittsburgh, PA and graduated in 2013 with a BA in Math and a BS in Math Education. I want to use evaluative methods that make children's experience learning in math the best it can be. I hope to form a researcher's perceptive in addition to an educator's perspective on quantitative methods that inform many decisions in schools. While enrolled part time in graduate courses, I am currently teaching middle school math at an independent school in Washington, DC.

Nathan Quimpo
M.S. student
nquimpo@umd.edu
I am a Master’s student in the QMMS program. In 2022, I graduated from New York University Abu Dhabi with a B.A. in Psychology with minors in Philosophy and Natural Science. Currently, my methodological interests lie in causal inference and my applied interests lie in the social determinants of population health disparities.
Brennan Register
Ph.D. student
brr@umd.edu
I am a third year Ph.D. student in the QMMS program. I earned my bachelor’s in Mathematics at Lock Haven University of Pennsylvania and my master’s degree in Statistics at The University of Pittsburgh before joining the QMMS program here at The University of Maryland.
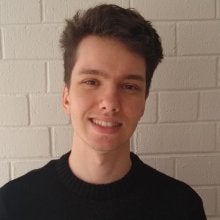
Patrick Sheehan
Ph.D. student
psheehan@umd.edu
In 2016, I graduated from The George Washington University with a bachelor's degree in psychology with a minor in statistics. In 2018, after working for two years, I joined EDMS as a master's student. During my time at QMMS, I have become very interested in delving deeper into topics in quantitative methodology, especially related to causal inference, and am very excited to be continuing my studies at QMMS as a Ph.D. student.

Joshua Shulkin
Ph.D. student
jshulkin@umd.edu
Before becoming a Ph.D. student in the QMMS program, I obtained my B.A. in Psychology with a minor in Politcal Science, and my M.A. in Psychology at the University of Colorado Colorado Springs. The range of statistics and methods courses I took during my M.A. fostered my interest in continuing my education in quantitative methods, particularly related latent variables and structural equation modeling
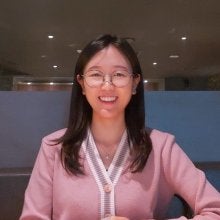
Youjin Sung
Ph.D. student
yjsung@umd.edu
Before joining the program, I graduated from Yonsei University, South Korea, with a bachelor's degree in Education and Applied Statistics and a master's degree in Educational Measurement. My current research interests include developing and applying latent variable models, focusing on item response theory and factor analysis.

Apoorva Verma
M.S. student
vermaa@umd.edu
I received my B.S. in Psychology from Hollins University, Virginia, and pursued a year of graduate coursework in Social Psychology at Arizona State University. My quantitative courses and research experiences, e.g., studying the measurement of trust in automated systems, piqued my interest in algorithmic fairness, educational equity, and machine learning. As a QMMS master's student, my research interests lie at the intersection of social network analysis, causal inference, and machine learning.
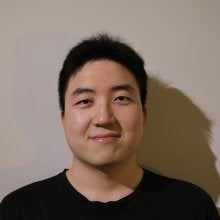
Hongyu Yang
M.S. student
hyang3@umd.edu
I graduated in 2018 with an M.S. in Economics from UIUC. When I knew the EDMS program by chance, I realized this is quite interesting and I would like to move forward with it. Currently, I am interested in statistical computing and latent variable modeling.

Jiaying Ye
Ph.D. student
tigerye@umd.edu
I was a Master of Science graduate student in the Applied Statistics program at the University of Michigan, Ann Arbor. I hold a Bachelor of Science in Statistics with a minor in Mathematics from North Carolina State University, Raleigh. I am excited to contribute to Quantitative Methods: Measurement and Statistics through research and collaboration.
Ph.D. Program
The Ph.D. program is designed to qualify individuals to teach courses at the university level in applied measurement, statistics, and evaluation, to provide leadership in the conduct of research studies, and to serve as applied statistics, measurement, or evaluation specialists in school systems, industry and government.
Courses within the program are selected from offerings of Quantitative Methodology: Measurement and Statistics (QMMS) and other departments of the University. A program for a student will be structured to take into account the background and future aims of the individual. There is a common core of courses comprised of (note new abbreviation QMMS, replacing EDMS):
QMMS 623: Applied Measurement: Issues and Practices (3)
QMMS 626: Instrumentation (3)
QMMS 646: General Linear Models I (3)
QMMS 647: Causal Inference and Evaluation Methods (3)
QMMS 651: General Linear Models II (3)
QMMS 655: Introduction to Multilevel Modeling (3)
QMMS 657: Exploratory Latent and Composite Variable Methods (3)
QMMS 722: Structural Modeling (3)
QMMS 724: Modern Measurement Theory (3)
QMMS 779: Mathematical Foundations and Simulation Techniques (3)
QMMS 787: Bayesian Inference and Analysis (3)
QMMS 899: Doctoral Dissertation Research (12)
In addition, at least 21 credits of elective courses must be selected in consultation with the student's advisor. A minimum of 30 credit hours (including QMMS 899) must be taken following admission. In addition to courses, students must complete a doctoral preliminary examination and a doctoral comprehensive examination and are expected to participate in research and publication. A faculty advisor may require courses beyond those specified in this document. The QMMS Program does not offer the Doctor of Education (Ed.D.) degree.
The many and varied professional interests of faculty members provide opportunities for presentations of research reports by students as well as faculty. Recent presentations by students have dealt with a variety of original research topics, in such areas as applied statistics, modeling of traits, test construction, test evaluation, and survey research.
M.S. Program
The M.S. program is designed to provide balanced intermediate level graduate training in quantitative methods for students preparing for a variety of positions in government, educational institutions, and private industry. Proximity to Washington, D.C., provides opportunities for students to engage in a variety of academic and professional experiences.
The program requires a minimum of 30 credit hours in courses acceptable for credit toward a graduate degree. At least 18 must be selected from courses numbered 600 or above, combining content from statistics, measurement, evaluation, and related fields outside of the program (a maximum of 6 outside hours).
Courses within the program are selected from offerings of the Quantitative Methodology: Measurement and Statistics program and other departments of the University. A program for a student will be structured to take into account the background and future aims of the individual. There is a common core of courses comprised of:
QMMS 623: Applied Measurement: Issues and Practices (3)
QMMS 646: General Linear Models I (3)
QMMS 647: Causal Inference and Evaluation Methods (3)
QMMS 651: General Linear Models II (3)
QMMS 655: Introduction to Multilevel Modeling (3)
QMMS 657: Exploratory Latent and Composite Variable Methods (3)
QMMS 724: Modern Measurement Theory (3)
Additional elective coursework completes the 30 credit hours. A written comprehensive examination based on the first four courses of the core (646, 651, 623, 647) is required. The Graduate School allows transfer of up to six credits of appropriate prior graduate work. Both thesis and non-thesis options are available in the Master of Science program. All coursework applied toward completion of the degree must be completed in a five year period. The program does not offer the Master of Education degree
Combined B.A. and M.S. program
The Quantitative Methodology: Measurement and Statistics (QMMS) program in the College of Education offers a 5th Year M.S. program for undergraduates interested in quantitative methods. This allows highly motivated undergraduates the chance to develop their skills in quantitative methods and complete both the bachelor's and master's degrees in approximately 5 years. This degree prepares one for careers that include statistical data analysis, developing survey and testing instruments, designing research studies, performing quantitative analysis, evaluating educational programs, psychometric analysis of assessment results, conducting marketing studies, and conducting surveys.
Almost any undergraduate major would be appropriate for this program, including psychology, sociology, mathematics, statistics, computer science, communications, business, and economics, and even English and history. The critical quality that a student needs to bring to the department is high quantitative ability. This is a profession that trains at the graduate level, and the specific undergraduate major is not a critical factor. Fields involving the application of modern quantitative research techniques provide an outlet for those trained in this program.
While an undergraduate, students can take three of the following four courses: EDMS646, EDMS651, EDMS623, EDMS647. These 9 credits would count toward both the undergraduate and graduate degree program. Students would need to also formally apply to the master’s program in their senior year. Please contact the EDMS Director of Graduate Studies for more information on the enrollment process.
Certificate
Students who are enrolled in another doctoral program at the University of Maryland can opt to apply to enter our certificate program. This program allows students to obtain skills and knowledge to have a quantitative specialization that they may use with their content area of interest. The certificate must be completed on or before the end of the doctoral degree; it requires completion of 21 credits, including the following courses:
QMMS 623: Applied Measurement: Issues and Practices (3)
QMMS 646: General Linear Models I (3)
QMMS 647: Causal Inference and Evaluation Methods (3)
QMMS 651: General Linear Models II (3)
The courses to be taken for the remaining nine credits should be chosen in consultation with the QMMS advisor for the student. For more information about the enrollment process for the certificate, please contact the Director of Graduate Studies for the EDMS program.
QMMS 451: Introduction to Educational Statistics (3 credits). Restriction: Sophomore standing or higher. Credit only granted for: BIOM301, BMGT230, CCJS200, ECON230, ECON321, QMMS451, GEOG306, GEOL351, GVPT422, PSYC200, or SOCY201. Introduction to statistical reasoning; location and dispersion measures; computer applications; regression and correlation; formation of hypotheses tests; t-test; one-way analysis of variance; analysis of contingency tables.
QMMS 489: Field Experiences in Measurement and Statistics (1-4 credits). Restriction: Permission of EDUC-Human Development and Quantitative Methodology department. Repeatable to 4 credits. Planned field experience in education-related activities. Credit not to be granted for experiences accrued prior to registration.
QMMS 498: Special Problems in Measurement and Statistics (1-3 credits). Prerequisite: Available only to education majors who have formal plans for individual study of approved problems. Restriction: Permission of EDUC-Human Development and Quantitative Methodology department. Repeatable to 6 credits. Available only to education majors who have formal plans for individual study of approved problems.
QMMS 610: Classroom Assessment and Evaluation (3 credits). Develop the understandings and skills needed to validly, reliably, and accurately assess student learning and to provide focused leadership in the area of classroom assessment.
QMMS 622: Theory and Practice of Standardized Testing (3 credits). Prerequisite: QMMS451; or QMMS645. Principles of interpretation and evaluation of aptitude, achievement, and personal-social instruments; theory of reliability and validity; prediction and classification; norm- and criterion-referenced testing concepts.
QMMS 623: Applied Measurement: Issues and Practices (3 credits). Prerequisite: QMMS645; or students who have taken courses with comparable content may contact the department. Measurement theory and its application at an intermediate level; test development, validation and interpretation; issues and recent developments in measurement.
QMMS 626: Instrumentation (3 credits). Prerequisite: QMMS623. Theory, development, and applications of various affective, cognitive, or behavioral measurement instruments and procedures, including questionnaire and test items, observational protocols, and cutting-edge innovative game and scenario-based assessments.
QMMS 645: Quantitative Research Methods I (3 credits). Research design and statistical applications in educational research: data representation; descriptive statistics; estimation and hypothesis testing. Application of statistical computer packages is emphasized.
QMMS 646: General Linear Models I (3 credits). Prerequisite: QMMS645; or an equivalent introductory statistics course. A first post-introductory inferential statistics course, with emphasis on analysis of variance procedures and designs from within the general linear modeling framework. Assignments include student analysis of education and related data; application of statistical software packages is emphasized.
QMMS 647: Causal Inference and Evaluation Methods (3 credits). Prerequisite: Must have completed or be concurrently enrolled in QMMS651. Counterfactual (potential outcomes) framework for causal inference, design/analysis strategies for confounder control, and specific best-practice applications to the evaluation of programs.
QMMS 651: General Linear Models II (3 credits). Prerequisite: QMMS646; or students who have taken courses with comparable content may contact the department. Multiple regression and correlation analysis; trend analysis; hierarchical and stepwise procedures; logistic regression; software for regression analysis.
QMMS 655: Introduction to Multilevel Modeling (3 credits). Prerequisite: QMMS651; or students who have taken courses with comparable content may contact the department. Introduction to multilevel models and methodology as strategies for modeling change and organizational effects.
QMMS 657: Exploratory Latent and Composite Variable Methods (3 credits). Prerequisite: EDMS651. Development of models for exploratory factor analysis and their practical applications. Additional topics will draw from latent class analysis, cluster analysis, mixture models, machine learning, multidimensional scaling, and configural frequency analysis.
QMMS 665: Data Analysis and Statistical Consulting (3 credits). Prerequisite: QMMS651; or students who have taken courses with comparable content may contact the department. Advanced data analysis procedures applied to real-world clients' problems arising in a wide variety of substantive research settings within and beyond education.
QMMS 722: Structural Modeling (3 credits). Prerequisite: EDMS657. Statistical theory and methods of estimation used in structural modeling; computer program applications; multisample models; mean structure models; structural models with multilevel data (e.g., sampling weights, growth models, multilevel latent variable models).
QMMS 724: Modern Measurement Theory (3 credits). Prerequisite: QMMS623 and QMMS651. Theoretical formulations of measurement from a latent trait theory perspective.
QMMS 738: Seminar in Special Problems in Measurement (1-3 credits). Restriction: Permission of EDUC-Human Development and Quantitative Methodology department. Repeatable to 3 credits. An opportunity for students with special interests to focus in depth on contemporary topics in measurement. Topics to be announced, but will typically be related to applied and theoretical measurement. Recent topics include: Large Scale Assessment, Advanced Item Response Theory, Computer Adaptive Testing
QMMS 747: Design of Program Evaluations (3 credits). Prerequisite: QMMS626, QMMS651, and QMMS647. Or permission of instructor; and permission of EDUC-Human Development and Quantitative Methodology department. Analysis of measurement and design problems in program evaluations.
QMMS 769: Special Topics in Applied Statistics in Education (1-4 credits). Restriction: Permission of EDUC-Human Development and Quantitative Methodology department. Designed primarily for students majoring or minoring in measurement, statistics or evaluation. Recent topics include: Mixture Models, Longitudinal Data Analysis, Advanced Structural Equation Modeling, Nonparametric Structural Equation Modeling
QMMS 779: Seminar in Applied Statistics: Mathematical Foundations and Simulation Techniques (3 credits). Restriction: Permission of EDUC-Human Development and Quantitative Methodology department. And must be in Quantitative Methodology: Measurement and Statistics Master's or Doctoral program. One part of the course will be dedicated to learning about simulation design, implementation, execution, and dissemination of results. For this material, SAS and R will be the primary computing platforms for both course delivery and student work. The second part of the course will include an introduction into statistical computing. This course material will be delivered in R and will involve using and writing code in R and/or SAS.
QMMS 787: Bayesian Inference and Analysis (3 credits). Prerequisite: QMMS651. Credit only granted for: QMMS769B or QMMS787. Formerly: QMMS769B. Models and model fitting methods commonly used in Bayesian Inference, such as Markov Chain Monte Carlo methods (e.g., Gibbs, Metropolis Sampling), with applications within and beyond the social and behavioral sciences. Analytical and philosophical differences between Frequentist and Bayesian statistics will also be highlighted.
QMMS 798: Special Problems in Education (1-6 credits). Restriction: Must be in Quantitative Methodology: Measurement and Statistics Master's or Doctoral program. Master's or doctoral students who desire to pursue special research problems under the direction of their advisors may register for credit under this number.
QMMS 799: Master's Thesis Research (1-6 credits). Restriction: Must be in a major within EDUC-Human Development and Quantitative Methodology department. Registration required to the extent of 6 credits.
QMMS 879: Doctoral Seminar (1-3 credits). Restriction: Permission of EDUC-Human Development and Quantitative Methodology department. Seminar that supports analysis of doctoral projects and theses, and of other on-going research projects.
QMMS 889: Internship in Measurement and Statistics (3-12 credits). Prerequisite: Open only to students advanced to candidacy for doctoral degree. Restriction: Permission of EDUC-Human Development and Quantitative Methodology department. Provides internship experiences at a professional level of competence in a particular role with appropriate supervision. Credit not to be granted for experience accrued prior to registration. Open only to students advanced to candidacy for doctoral degree.
QMMS 898: Pre-Candidacy Research (1-8 credits)
QMMS 899: Doctoral Dissertation Research (1-8 credits). Registration required to the extent of 12 credits.
QMMS Psychometric Computation and Simulation Lab
The QMMS Psychometric Computation and Simulation (PCS) lab provides QMMS students with state-of-the-art software resources to conduct advanced cutting-edge simulation and computational research, and provides students and faculty a means to conduct highly specialized training sessions for students and researchers from across our campus and from other institutions who are seeking high level methodological training.
Maryland Assessment Research Center (MARC)
The Maryland Assessment Research Center (MARC) provides support to the range of assessment activities in the State, the region and nation by conducting basic and applied research to enhance the quality of assessment practice and knowledge. MARC is a project of the Quantitative Methodology: Measurement and Statistics (QMMS) program in the Department of Human Development and Quantitative Methodology in the College of Education at the University of Maryland.
To accomplish its purposes, MARC houses expertise in assessment design, development, implementation, analysis, reporting and policy issues as well as the technical aspects of the quantitative theories that form the foundations of measurement. The nationally recognized EDMS program in the Department of Human Development and Quantitative Methodology, College of Education, and College Park Campus of the University of Maryland (UMCP) augment the capabilities of the MARC staff.
Center for Integrated Latent Variable Research (CILVR)
CILVR is a center whose goal is to serve as a national and international focal point for innovative collaboration, state-of-the-art training, and scholarly dissemination as they relate to the full spectrum of latent variable statistical methods.
CILVR is housed within the Quantitative Methodology: Measurement and Statistics (QMMS) program at the University of Maryland. QMMS faculty are recognized scholars in various facets of latent variable statistical models, whether it be item response theory, latent class analysis, mixture models, or structural equation modeling. QMMS is also unique in its geographic location, situated along the East Coast of the United States near the nation's capital, centrally located for scholars from the US and Europe, and a short distance from some of the world's top latent variable scholars working in academia, government, and applied research settings. Thus, QMMS is well positioned in its composition and its location to serve as a focal point for integrated latent variable research.
Here is a link to our catalog: https://umd-cilvr.catalog.instructure.com/
DATES | EVENT/TOPIC | INSTRUCTOR | RATES | MORE INFO | |||||||||||||||||||||||||||||||||||||||||||||||||||||||||||||||||||||||||||||||||||||||||||||||||||||||||||||||||||||||||||||||||||||||||||||||||||||||||||||||||||||||||||||||||||||||||||||||||||||||||||||||||||||||||||||||||||||||||||||||||||||||||||||||||||||||||||||||||||||||||||||||||||||||||||||||||||||||||||||||||||||||||||||||||||||||||||||||||||||||||||||||||||||||||||||||||||||||||||||||||||||||||||||||||||||||||||||||||||||||||||||||||||||||||||||||||||||||||||||||||||||||||||||||||||||||||||||||||||||||||||||||||||||||||||||||||||||||||||||||||||||||||||||||||||||||||||||||||||||||||||||||||||||||||||||||||||||||||||||||||||||||||||||||||||||||||||||||||||||||||||||||||||||||||||||||||||||||||||||||||||||||||||||||||||||||||||||||||||||||||||||||||||||||||||||||||||||||||||||||||||||||||||||||||||||||||||||||||||||||||||||||||||||||||||||||||||||||||||||||||||||||||||||||||||||||||||||||||||||||||||||||||||||||||||||||||||||||||||||||||||||||||||||||||||||||||||||||||||
November 7-8 2024 (Thu-Fri) | Analysis of Complex Survey Data (from NCES) ONLINE | Dr. Laura Stapleton, University of Maryland | Full-time student: $195 Professional: $375 | https://umd-cilvr.catalog.instructure.com/courses/analysis-of-complex-survey-data-from-nces-2024 | |||||||||||||||||||||||||||||||||||||||||||||||||||||||||||||||||||||||||||||||||||||||||||||||||||||||||||||||||||||||||||||||||||||||||||||||||||||||||||||||||||||||||||||||||||||||||||||||||||||||||||||||||||||||||||||||||||||||||||||||||||||||||||||||||||||||||||||||||||||||||||||||||||||||||||||||||||||||||||||||||||||||||||||||||||||||||||||||||||||||||||||||||||||||||||||||||||||||||||||||||||||||||||||||||||||||||||||||||||||||||||||||||||||||||||||||||||||||||||||||||||||||||||||||||||||||||||||||||||||||||||||||||||||||||||||||||||||||||||||||||||||||||||||||||||||||||||||||||||||||||||||||||||||||||||||||||||||||||||||||||||||||||||||||||||||||||||||||||||||||||||||||||||||||||||||||||||||||||||||||||||||||||||||||||||||||||||||||||||||||||||||||||||||||||||||||||||||||||||||||||||||||||||||||||||||||||||||||||||||||||||||||||||||||||||||||||||||||||||||||||||||||||||||||||||||||||||||||||||||||||||||||||||||||||||||||||||||||||||||||||||||||||||||||||||||||||||||||||||
December 12-13, 2024 (Thu-Fri) | Multilevel Modeling ONLINE | Dr. Tracy Sweet, University of Maryland | Full-time student: $195 Professional: $375 | https://umd-cilvr.catalog.instructure.com/courses/multilevel-modeling-2024 | |||||||||||||||||||||||||||||||||||||||||||||||||||||||||||||||||||||||||||||||||||||||||||||||||||||||||||||||||||||||||||||||||||||||||||||||||||||||||||||||||||||||||||||||||||||||||||||||||||||||||||||||||||||||||||||||||||||||||||||||||||||||||||||||||||||||||||||||||||||||||||||||||||||||||||||||||||||||||||||||||||||||||||||||||||||||||||||||||||||||||||||||||||||||||||||||||||||||||||||||||||||||||||||||||||||||||||||||||||||||||||||||||||||||||||||||||||||||||||||||||||||||||||||||||||||||||||||||||||||||||||||||||||||||||||||||||||||||||||||||||||||||||||||||||||||||||||||||||||||||||||||||||||||||||||||||||||||||||||||||||||||||||||||||||||||||||||||||||||||||||||||||||||||||||||||||||||||||||||||||||||||||||||||||||||||||||||||||||||||||||||||||||||||||||||||||||||||||||||||||||||||||||||||||||||||||||||||||||||||||||||||||||||||||||||||||||||||||||||||||||||||||||||||||||||||||||||||||||||||||||||||||||||||||||||||||||||||||||||||||||||||||||||||||||||||||||||||||||||
January 8-10, 2025 (Wed-Fri) | Structural Equation Modeling: From Beginner to Intermediate ONLINE | Dr. Gregory R. Hancock, University of Maryland with Dr. Yi Feng, UCLA | Full-time student: $295 Professional: $525 | https://umd-cilvr.catalog.instructure.com/courses/structural-equation-modeling-from-beginner-to-intermediate-2025 | |||||||||||||||||||||||||||||||||||||||||||||||||||||||||||||||||||||||||||||||||||||||||||||||||||||||||||||||||||||||||||||||||||||||||||||||||||||||||||||||||||||||||||||||||||||||||||||||||||||||||||||||||||||||||||||||||||||||||||||||||||||||||||||||||||||||||||||||||||||||||||||||||||||||||||||||||||||||||||||||||||||||||||||||||||||||||||||||||||||||||||||||||||||||||||||||||||||||||||||||||||||||||||||||||||||||||||||||||||||||||||||||||||||||||||||||||||||||||||||||||||||||||||||||||||||||||||||||||||||||||||||||||||||||||||||||||||||||||||||||||||||||||||||||||||||||||||||||||||||||||||||||||||||||||||||||||||||||||||||||||||||||||||||||||||||||||||||||||||||||||||||||||||||||||||||||||||||||||||||||||||||||||||||||||||||||||||||||||||||||||||||||||||||||||||||||||||||||||||||||||||||||||||||||||||||||||||||||||||||||||||||||||||||||||||||||||||||||||||||||||||||||||||||||||||||||||||||||||||||||||||||||||||||||||||||||||||||||||||||||||||||||||||||||||||||||||||||||||||
January 15-17, 2025 (Wed-Fri) | Introduction to Longitudinal Structural Equation and Latent Growth Modeling ONLINE | Dr. Gregory R. Hancock, University of Maryland with Dr. Yi Feng, UCLA | Full-time student: $295 Professional: $525
| https://umd-cilvr.catalog.instructure.com/courses/introduction-to-longitudinal-structural-equation-and-latent-growth-modeling-2025 | |||||||||||||||||||||||||||||||||||||||||||||||||||||||||||||||||||||||||||||||||||||||||||||||||||||||||||||||||||||||||||||||||||||||||||||||||||||||||||||||||||||||||||||||||||||||||||||||||||||||||||||||||||||||||||||||||||||||||||||||||||||||||||||||||||||||||||||||||||||||||||||||||||||||||||||||||||||||||||||||||||||||||||||||||||||||||||||||||||||||||||||||||||||||||||||||||||||||||||||||||||||||||||||||||||||||||||||||||||||||||||||||||||||||||||||||||||||||||||||||||||||||||||||||||||||||||||||||||||||||||||||||||||||||||||||||||||||||||||||||||||||||||||||||||||||||||||||||||||||||||||||||||||||||||||||||||||||||||||||||||||||||||||||||||||||||||||||||||||||||||||||||||||||||||||||||||||||||||||||||||||||||||||||||||||||||||||||||||||||||||||||||||||||||||||||||||||||||||||||||||||||||||||||||||||||||||||||||||||||||||||||||||||||||||||||||||||||||||||||||||||||||||||||||||||||||||||||||||||||||||||||||||||||||||||||||||||||||||||||||||||||||||||||||||||||||||||||||||||
February 14, 2025 (Fri) | Item Writing and Questionnaire Design ONLINE | Dr. Laura Stapleton, University of Maryland | Full-time student: $95 Professional: $225 | https://umd-cilvr.catalog.instructure.com/courses/item-writing-and-questionnaire-design-2025 | |||||||||||||||||||||||||||||||||||||||||||||||||||||||||||||||||||||||||||||||||||||||||||||||||||||||||||||||||||||||||||||||||||||||||||||||||||||||||||||||||||||||||||||||||||||||||||||||||||||||||||||||||||||||||||||||||||||||||||||||||||||||||||||||||||||||||||||||||||||||||||||||||||||||||||||||||||||||||||||||||||||||||||||||||||||||||||||||||||||||||||||||||||||||||||||||||||||||||||||||||||||||||||||||||||||||||||||||||||||||||||||||||||||||||||||||||||||||||||||||||||||||||||||||||||||||||||||||||||||||||||||||||||||||||||||||||||||||||||||||||||||||||||||||||||||||||||||||||||||||||||||||||||||||||||||||||||||||||||||||||||||||||||||||||||||||||||||||||||||||||||||||||||||||||||||||||||||||||||||||||||||||||||||||||||||||||||||||||||||||||||||||||||||||||||||||||||||||||||||||||||||||||||||||||||||||||||||||||||||||||||||||||||||||||||||||||||||||||||||||||||||||||||||||||||||||||||||||||||||||||||||||||||||||||||||||||||||||||||||||||||||||||||||||||||||||||||||||||||
February 28 - March 1, 2025 (Fri-Sat) | Introduction to Meta-Analysis ONLINE | Dr. Joshua Polanin, American Institutes of Research | Full-time student: $195 Professional: $375 | https://umd-cilvr.catalog.instructure.com/courses/introduction-to-meta-analysis-2025 | |||||||||||||||||||||||||||||||||||||||||||||||||||||||||||||||||||||||||||||||||||||||||||||||||||||||||||||||||||||||||||||||||||||||||||||||||||||||||||||||||||||||||||||||||||||||||||||||||||||||||||||||||||||||||||||||||||||||||||||||||||||||||||||||||||||||||||||||||||||||||||||||||||||||||||||||||||||||||||||||||||||||||||||||||||||||||||||||||||||||||||||||||||||||||||||||||||||||||||||||||||||||||||||||||||||||||||||||||||||||||||||||||||||||||||||||||||||||||||||||||||||||||||||||||||||||||||||||||||||||||||||||||||||||||||||||||||||||||||||||||||||||||||||||||||||||||||||||||||||||||||||||||||||||||||||||||||||||||||||||||||||||||||||||||||||||||||||||||||||||||||||||||||||||||||||||||||||||||||||||||||||||||||||||||||||||||||||||||||||||||||||||||||||||||||||||||||||||||||||||||||||||||||||||||||||||||||||||||||||||||||||||||||||||||||||||||||||||||||||||||||||||||||||||||||||||||||||||||||||||||||||||||||||||||||||||||||||||||||||||||||||||||||||||||||||||||||||||||||
March 27-28, 2025 (Thu-Fri) | Applied Causal Inference with Directed Acyclic Graphs ONLINE | Dr. Peter Steiner, University of Maryland | Full-time student: $195 Professional: $375 | https://umd-cilvr.catalog.instructure.com/courses/applied-causal-inference-with-directed-acyclic-graphs | |||||||||||||||||||||||||||||||||||||||||||||||||||||||||||||||||||||||||||||||||||||||||||||||||||||||||||||||||||||||||||||||||||||||||||||||||||||||||||||||||||||||||||||||||||||||||||||||||||||||||||||||||||||||||||||||||||||||||||||||||||||||||||||||||||||||||||||||||||||||||||||||||||||||||||||||||||||||||||||||||||||||||||||||||||||||||||||||||||||||||||||||||||||||||||||||||||||||||||||||||||||||||||||||||||||||||||||||||||||||||||||||||||||||||||||||||||||||||||||||||||||||||||||||||||||||||||||||||||||||||||||||||||||||||||||||||||||||||||||||||||||||||||||||||||||||||||||||||||||||||||||||||||||||||||||||||||||||||||||||||||||||||||||||||||||||||||||||||||||||||||||||||||||||||||||||||||||||||||||||||||||||||||||||||||||||||||||||||||||||||||||||||||||||||||||||||||||||||||||||||||||||||||||||||||||||||||||||||||||||||||||||||||||||||||||||||||||||||||||||||||||||||||||||||||||||||||||||||||||||||||||||||||||||||||||||||||||||||||||||||||||||||||||||||||||||||||||||||||
May 15-16, 2025 (Thu-Fri) | Introduction to Social Network Analysis ONLINE | Dr. Tracy Sweet, University of Maryland | Full-time student: $195 Professional: $375 | https://umd-cilvr.catalog.instructure.com/courses/introduction-to-social-network-analysis-2025 | |||||||||||||||||||||||||||||||||||||||||||||||||||||||||||||||||||||||||||||||||||||||||||||||||||||||||||||||||||||||||||||||||||||||||||||||||||||||||||||||||||||||||||||||||||||||||||||||||||||||||||||||||||||||||||||||||||||||||||||||||||||||||||||||||||||||||||||||||||||||||||||||||||||||||||||||||||||||||||||||||||||||||||||||||||||||||||||||||||||||||||||||||||||||||||||||||||||||||||||||||||||||||||||||||||||||||||||||||||||||||||||||||||||||||||||||||||||||||||||||||||||||||||||||||||||||||||||||||||||||||||||||||||||||||||||||||||||||||||||||||||||||||||||||||||||||||||||||||||||||||||||||||||||||||||||||||||||||||||||||||||||||||||||||||||||||||||||||||||||||||||||||||||||||||||||||||||||||||||||||||||||||||||||||||||||||||||||||||||||||||||||||||||||||||||||||||||||||||||||||||||||||||||||||||||||||||||||||||||||||||||||||||||||||||||||||||||||||||||||||||||||||||||||||||||||||||||||||||||||||||||||||||||||||||||||||||||||||||||||||||||||||||||||||||||||||||||||||||||
The Quantitative Methodology: Measurement and Statistics (QMMS) program at the University of Maryland is continually seeking qualified students for its master's and doctoral level programs.
We offer:
- One of the nation's best programs in quantitative methods.
- A large number of Research and Teaching Assistantships.
- Training in a field with great career potential.
- Opportunities for field work on campus, in the Washington area, and nationally.
- A capable and active group of graduate students.
- Opportunities to work on projects through grants, contracts, and research centers.
What are Measurement, Statistics, and Evaluation?
Measurement: At its most basic level, the field of Measurement is concerned with the assignment of numbers to objects in some systematic, meaningful way. In education and the social sciences, measurement typically refers to a rational assignment of numbers so that they quantitatively describe some unobservable (i.e., latent) construct like ability, personality, attitude, satisfaction, etc. The assignment of these numbers must proceed in a carefully prescribed, reproducible fashion. In most cases, the process is based on a mathematical model that defines how the numbers should behave as the underlying construct changes. Measurement professionals develop and utilize a variety of mathematical tools to determine the reliability, validity and meaning of the numbers assigned during the measurement process. They are employed in both research and applied settings that involve psychological testing, educational testing, or the measurement of attitudes and preferences. These include large scale testing programs like the SAT and ACT.
Statistics: The field of Applied Statistics is primarily concerned with the development of testable research hypotheses, the application of powerful statistical tests to determine the plausibility of a given research hypothesis, and the design of experiments to control extraneous sources of variation. Other facets of this discipline include the design of schemes to collect sample data that are representative of a given population, the description of a population using alternative characteristics of sample data, and the development of alternative models to explain relationships between observable variables. Additionally, the assessment of model fit and the estimation of model parameters also fall under the rubric of Applied Statistics. Professionals in Applied Statistics develop and utilize quantitative techniques to test hypotheses, develop models for observed data, and assess the adequacy of those models. They are employed in a variety of settings where practical decisions must be made on the basis of observed data (e.g., business, industry, government, and education). These include agencies like the American Institutes of Research, the U.S. Census Bureau, the National Center for Education Statistics, and a large number of private organizations that conduct surveys or polls.
Evaluation: The field of Evaluation is concerned with the application of measurement and statistical principles to objectively evaluate institutional and organizational programs. Programs are generally evaluated with regard to the way in which they are planned and implemented, and the degree to which they accomplish their mission. Examples of such evaluation efforts include the Tennessee model for evaluating school effectiveness, the extensive work on the effects of teacher training upon student success, and the evaluations of the National Head Start Program. Evaluation professionals might work for the Federal Government as contract monitors, for an industry interested in determining their level of success in marketing, sales, or production, for the State Superintendent of Schools examining the results of large expenditures of time and money, and of course, for a University interested in teaching and research in the systematic application of measurement and statistics to the determination of program value.
The Programs
Quantitative Methodology: Measurement and Statistics (QMMS) offers programs of study leading to both the Master of Science (M.S.) and Doctor of Philosophy (Ph.D.) degrees. M.S. students generally take introductory coursework in measurement, applied statistics, and evaluation. Ph.D. students typically complete an analogous program of study, after which they may continue with a multidisciplinary focus or concentrate primarily on a single discipline.
Most of the educational and social science research that takes place today relies on the expertise of those who develop data collection instruments (such as assessments, questionnaires, and interview protocols), plan research and evaluation studies, develop new models and methods, design sampling frameworks, and collect and analyze data. The University of Maryland programs in Quantitative Methodology: Measurement and Statistics provide students with advanced skills in these areas. The master's program gives individuals the broad range of skills necessary to serve as research associates in academic, government, and business settings. The doctoral program qualifies individuals to provide leadership in the conduct of research studies, to serve as applied statisticians, measurement, or evaluation specialists in school systems, industry, and government, and to teach quantitative courses at the university level. We are widely recognized as one of the best applied quantitative programs in the country. Our students are exceptional. Our faculty members are respected leaders within their specialties.
Assistantships
We can usually offer research or teaching assistantships to most of our full-time students. Assistantships are full-time appointments (20 hours per week) that carry both a stipend and a tuition waiver. During a research assistantship, students pursue both individual and collaborative research projects with a faculty member advisor. These appointments are renewable. The duties of teaching assistants range from instructional support (e.g., grading) to taking full responsibility for teaching a section of an undergraduate course (with appropriate supervision and support).
Career Opportunities
There is a tremendous demand for individuals with the quantitative skills provided by our program. Recent surveys of degree programs and employment in measurement show that there will continue to be a shortfall in the number of measurement professionals relative to the number of available employment opportunities. Similarly, the National Science Foundation predicts a strong job market for statisticians over the next ten years.
The need for applied quantitative professionals is strikingly evident in educational research. As education in the United States pushes toward greater accountability and better informed decision making, there has been an ever-increasing demand for professionals who can interpret the abundance of collected data. Each of the nation's 16,000 school districts and each of the 50 state education departments collects large quantities of data to assess the impact of schooling in its community. There are many state and federal data-collection programs, ranging from a regular biennial survey of U.S. schools to specialized cross-sectional and longitudinal surveys. Many of these survey and testing programs result from legislative mandates and they all rely heavily on instrumentation, research design, sampling schemes, and data analysis. Almost all educational research today depends on hard data, rigorous quantitative approaches, and individuals with research expertise. This is a field that is expanding; people recognize that quantitative methods are critical to developing educational theory and are needed to provide a sound foundation for educational progress. As summarized by the American Statistical Association, [People with quantitative skills] concerned with educational issues readily find employment in universities, state and federal government agencies, and private research agencies. The many needs of the education sector for statistical expertise promise exciting careers to all whose interests lie in this field.
Quantitative professionals are not only in high demand, but they also have jobs that are both professionally and personally desirable. The problems that these professionals help solve often have substantial impact on the lives of many other individuals. Additionally, many sources often rank the job of "Statistician" as the most attractive profession with regard to working conditions.
Field Work and Postgraduate Opportunities
Our reputation and location provide tremendous opportunities for our students to conduct special projects and be involved with real-world, often ground-breaking, applications in government, research firms, associations, and private industry. Current and recent students have conducted special projects with or have been employed by American Institutes for Research, The Substance Abuse and Mental Health Services Administration, The Census Bureau, Westat, State Departments of Education, Local Education Agencies, National Education Association, U.S. Department of Education, Educational Testing Service, Maryland Assessment Research Center, Center for Applied Linguistics, and the Center for Biologics Evaluation and Research of the Food and Drug Administration. They also have taken professorial positions at such institutions as Arizona State University, University of Nebraska, University of Minnesota, University of Georgia, University of Texas - Austin, The George Washington University, University of Houston, Southern Illinois University, University of Hawaii, and University of Maryland Baltimore County.
Student Body
We are committed to maintaining high standards for admission to both our master's and doctoral programs, and we have been able to attract top students from across the United States and the world. Our students come from undergraduate institutions, government, professional associations, consulting firms, research units of companies, and the public schools. Our doctoral students are among the best on any campus. The real-world experiences, skills, and aptitude of our students help make our program intellectually rigorous while providing exceptional peer-to-peer support. Approximately one-half of our students attend full-time, and there is a very active departmental student organization.
Frequently Asked Questions
How many students are in the program? We currently have approximately 35 students enrolled in our M.S. and Ph.D. programs.
Do I need to have experience in education to be accepted? No. Our students come from a variety of disciplines both within and outside of education. The dominant characteristic of successful candidates is that they are very interested in the application of quantitative techniques to solve practical problems. Many of these problems arise in educational settings, but others emerge in the social sciences, government, business, and industry.
I have an undergraduate degree (B.A./B.S.) - may I enter directly into the Ph.D. program? This is a possibility, but very rare. Typically, Ph.D. applicants first complete a relevant master's program. If one completes the QMMS master's program first, then one may apply for the Ph.D. program subsequently. Note that all courses taken as part of an QMMS master's degree apply toward the Ph.D., and the doctoral preliminary examination is waived for those who successfully complete the QMMS master's degree.
Do I need to have a background in mathematical statistics to enter your program? No, but strong quantitative skills and interests are necessary. Undergraduate courses in calculus and linear algebra provide necessary skills for our program.
Do you have many foreign students in your programs? Yes. Approximately 50% of our students are from countries other than the United States. For example, we have strong contingents of students from China and Korea.
What are the admissions criteria? The QMMS Admissions Committee meets regularly during the academic year. Decisions are based on several sources of information including scores on the Graduate Record Examination (GRE), undergraduate transcripts, letters of recommendation, and a statement of interest.
What are the deadlines for admission? Please see the Graduate School web site for current information about admissions for applicants including those holding foreign visas. Our selection committee meets throughout the academic year. However, note that the Graduate School clearance procedures for foreign students can take several months and we do not see your application until these clearances are complete. All applicants should keep track of their applications by visiting the Graduate School web site rather than by e-mailing QMMS.
How much is tuition? Please see the Bursar's Office web site for current information about student tuition. Note that students with full-time teaching or research assistantships, or fellowships, within the Department receive a tuition waiver in the amount of 10 credit hours per semester.
What types of financial aid are available? In addition to Department teaching and research assistantships, the University also offers financial assistance in the form of graduate fellowships, tuition scholarships, support grants, and variety of need-based financial aid programs. However, since financial aid is limited, those applicants who need financial aid should apply as early as possible.
When are classes held? Most classes are held once per week during the evening hours (e.g., 4:15-7:00 PM) so that part-time students with employment/family commitments can attend along with full-time students.
How long does it take a student to finish the program? The degree programs are structured so that a full-time student could finish requirements for the M.S. degree in approximately 2 years whereas approximately 4 years would be required to finish the Ph.D. requirements.
How well does our program accommodate part-time students? Most courses in the department are scheduled during late afternoon or evening hours to enable part-time students to attend classes. Faculty are generally available for consultation during evening hours as well. This provides part-time students with opportunities for both traditional instruction and individual study/research mentored by department faculty. However, some required courses for Ph.D. students require attendance during daytime hours.
More Information? Contact Dr. Gregory R. Hancock, ghancock@umd.edu, Program Director, EDMS
The University of Maryland is an equal opportunity institution with respect to both education and employment. The university's policies, programs and activities are in conformance with state and federal laws and regulations on non-discrimination regarding race, color, religion, age, national origin, political affiliation, gender, sexual orientation or disability
The College of Education has long provided instruction in quantitative research methods. Prior to 1964, there was one full-time professor in the area serving a College faculty of approximately 50 members. Although there were recognized areas of specialization, there was, at that time, no departmental structure within the College. Rather, the College operated as a single administrative unit headed by a Dean. During the next ten years there was rapid growth in the size of College, reaching about 200 faculty by the mid-1970s. In this period, several new departments were formed including EDMS around 1972 (we’re a little fuzzy on the exact year). Throughout its history as a recognized area within the College, as a department, and now as a program within the HDQM Department, QMMS (formerly EDMS) has engaged in the dual roles of professional training and service to the College.
In its first role, QMMS has provided training at the master's and doctoral levels for students planning to pursue careers in quantitative areas related to applied statistics, measurement, and evaluation. An important impetus for the development of the major program was a relatively large fellowship program funded in 1966 by Title IV of the Elementary and Secondary Education Act (ESEA). This grant provided support for 12 doctoral students per year with about half of the positions going to QMMS majors and the remainder to minors with majors in other departments within the College. Graduates from the original ESEA fellowship program pursued a variety of important career paths including professorships in higher education, research positions with Maryland boards of education and research posts in the federal government. In fact, one very early graduate went on to become superintendent of the Baltimore City school system. The major program in QMMS had, and still has, a total of about 40 full- and part-time students. The number of fulltime faculty in QMMS has fluctuated from six to eight with a budgeted complement of eight at present.
In its second role, QMMS has provided service courses in applied statistics, measurement, and evaluation for graduate students majoring in other departments and programs in the College. In addition, faculty members have always been in high demand as members of doctoral research committees and as consultants to various externally funded projects within the College. This latter activity dates to the very earliest days of QMMS (then EDMS) when one faculty member was partially funded by a grant in the area of pupil personnel services and counselor education. The College has always required some course work provided by QMMS, with courses now in high demand across the campus. The professional training and service roles of QMMS serve complementary purposes. Virtually all full-time graduate students in QMMS are on some form of financial support and many of these students play key roles in service courses. In particular, several QMMS students each semester are employed as teaching assistants (tutors) for students from other units who are enrolled in courses such as QMMS 451, QMMS 645, QMMS 646, and QMMS 651. Also, advanced graduate students serve as primary instructors for the undergraduate QMMS 451 course. In effect, a strong cadre of QMMS graduate students is central to the service teaching role of the department.
Historically, QMMS had not vigorously sought external funding for its own research efforts. While, as noted, faculty members have participated in projects in other units, the procurement of major funding was rarely pursued. However, within the last 20 years QMMS has, with great success, focused major effort in the procurement of external funding through both grants and contracts dedicated to efforts specifically in measurement, statistics, and evaluation.
Feng, Y., & Hancock, G. R. (in press). Power in structural equation modeling. In R. Hoyle (Ed.), Handbook of structural equation modeling (2nd ed.). Guilford Press.
Feng, Y., & Hancock, G. R. (in press). An SEM-based framework for modeling variability as a latent variable. Psychological Methods.
Feng, Y., & Hancock, G. R. (in press). Model-based incremental validity. Psychological Methods.
Curran, P. J., & Hancock, G. R. (2021). The challenge of modeling co-developmental processes over time. Child Development Perspectives, 15, 67-75.
Feng, Y., & Hancock, G. R. (2021). Oh no! They cut my funding! Using 'post hoc' planned missing data designs to salvage longitudinal research. Child Development, 92, 1199-1216.
Hancock, G. R., & An, J. (2020). A closed-form alternative for estimating ω reliability under unidimensionality. Measurement: Interdisciplinary Research and Perspectives, 18, 1-14.
Johnson, T. L., & Hancock, G. R. (2019). Time to criterion latent growth models. Psychological Methods, 24, 690-707.
Feng, Y., Hancock, G. R., & Harring, J. R. (2019). Latent growth models with fixed floors and ceilings. Multivariate Behavioral Research, 54, 1-20.
Bonnéry, D., Feng, Y., Henneberger, A. K., Johnson, T. L., Lachowicz, M., Rose, B. A., Shaw, T., Stapleton, L. M., Woolley, M. E., Zheng, Y. (2019). The promise and limitations of synthetic data as a strategy to expand access to state-level multi-agency longitudinal data. Journal of Research on Educational Effectiveness. [Note: authors listed in alphabetical order]
Stapleton, L. M., & Johnson, T. L. (2019). Models to examine the validity of cluster-level factor structure using individual-level data. Advances in Methods and Practices in Psychological Science, 2, 312-329.
Wang, W., Liao, M., & Stapleton, L. M. (2019). Incidental second-level dependence in educational survey data with a nested data structure. Educational Psychology Review, 31, 571-596.
Blozis, S. A., & Harring, J. R. (2018). Fitting nonlinear mixed-effects models with alternative residual covariance structures. Sociological Research & Methods.
Hancock, G. R., & An, J. (2018). Framing and improving scale reliability assessment using structural equation models. Educational Measurement: Issues and Practice.
Hancock, G. R., Harring, J. R., & Macready, G. B. (Eds.). (2019). Advances in latent class analysis: A Festschrift in honor of C. Mitchell Dayton. Charlotte, NC: Information Age Publishing.
Hancock, G. R., Stapleton, L. M., & Mueller, R. O. (Eds.). (2019). The reviewer's guide to quantitative methods in the social sciences (2nd ed.). New York: Routledge.
Harring, J. R., & Blozis, S. A. (in press). Modeling nonlinear longitudinal change with mixed effects models. In A. A. O’Connell & D. B. McCoach (Eds.), Multilevel modeling with introductory and advanced applications.
Harring, J. R., & Johnson, T. (2018). Two-way analysis of variance. In B. Frey (Ed.), The SAGE encyclopedia of educational research, measurement and evaluation. Thousand Oaks, CA: SAGE Publications.
Jiao, H., & Li, C. (2018). Progress in International Reading Literacy Study (PIRLS) data. In B. Frey (Ed.), The SAGE Encyclopedia of Educational Research, Measurement, and Evaluation. Thousand Oaks, CA: Sage.
Jiao, H., & Liao, D. (2018). Testlet response theory. In B. Frey (Ed.), The SAGE Encyclopedia of Educational Research, Measurement, and Evaluation. Thousand Oaks, CA: Sage.
Man, K., Harring, J., Jiao, H., & Zhan, P. (in press). Conditional joint modeling of compensatory multidimensional item responses and response times. Applied Psychological Measurement.
Jiao, H., & Lissitz, R. W. (2018, Eds.). Data analytics and psychometrics: Informing assessment practices. Charlotte, NC: Information Age Publishing.
Jiao, H., & Lissitz, R. W. (in press). Applications of artificial intelligence to assessment. Charlotte, NC: Information Age Publisher.
Jiao, H., Liao, D., & Zhan, P. (in press). Utilizing process data for cognitive diagnosis. In M. von Davier & Y. Lee (Eds.), Handbook of diagnostic classification models.
Lee, D., Harring, J. R., & Stapleton, L. M. (in press). Comparing methods for addressing missingness in longitudinal modeling of panel data. Journal of Experimental Education.
Leite, W. L., Stapleton, L. M., & Bettini, E. F. (2018). Propensity score analysis of complex survey data with structural equation modeling: A tutorial with Mplus. Structural Equation Modeling: A Multidisciplinary Journal.
Little, T. D., Widaman, K. F., Levy, R., Rodgers, J. L., & Hancock, G. R. (2018). Error, error in my model, who’s the fairest of them all? Research on Human Development, 14, 271-286.
Liu, J., & Harring, J. R. (in press). A systematic investigation of within-subject and between-subject covariance structures in growth mixture models. In G. R. Hancock, J. R. Harring, & G. B. Macready (Eds.). Advances in latent class analysis: A Festschrift in honor of C. Mitchell Dayton. Charlotte, NC: Information Age Publishing.
Man, K., Harring, J. R., Jiao, H., & Zahn, P. (in press). Joint modeling of compensatory multidimensional item responses and response times. Applied Psychological Measurement.
Man, K., Harring. J. R., Ouyang, U., & Thomas, S. L. (2018). Response time based nonparametric Kullback-Leibler divergence measure for detecting aberrant test-taking behavior. International Journal of Testing, 18, 155-177.
Man, K., Sinharay, S., & Harring, J. R. (in press). Use of data mining methods to detect test fraud. Journal of Educational Measurement.
McNeish, D., & Hancock, G. R. (2018). The effect of measurement quality on targeted structural model fit indices: A comment on Lance, Beck, Fan, and Carter (2016). Psychological Methods, 23, 184-190.
Mueller, R. O., & Hancock, G. R. (2019). Structural equation modeling. In G. R. Hancock & R. O. Mueller (Eds.), The reviewer's guide to quantitative methods in the social sciences (2nd ed.) (pp. 445-456). New York: Routledge.
Qiao, X., & Jiao, H. (2018). Comparing data mining techniques in analyzing process data: A case study on PISA 2012 problem-solving items. Frontiers in Psychology.
Stapleton, L. M. (2019). Survey sampling, administration, and analysis. In G. R. Hancock, L. M. Stapleton & R. O. Mueller (Eds.), The reviewer’s guide to quantitative methods in the social sciences (2nd ed.) (pp. 467-481). New York, NY: Routledge.
Stapleton, L. M., & Kang, Y. (2018). Design effects of multilevel estimates from national probability samples. Sociological Methods & Research, 47, 430-457.
Stapleton, L. M., & Thomas, S. L. (in press). Using national and international datasets in multilevel modeling. In A. O’Connell, B. McCoach, & B. Bell (Eds.), Multilevel modeling methods with introductory and advanced applications. Charlotte, NC: Information Age Publishing.
Stegmann, G., Jacobucci, R., Harring, J. R., & Grimm, K. J. (2018). Nonlinear mixed-effects modeling programs in R. Structural Equation Modeling: A Multidisciplinary Journal, 25, 160-165.
Sweet, T. M. (2019). Social network analysis. In G. R. Hancock, L. M. Stapleton, & R. O. Mueller (Eds.), The reviewer's guide to quantitative methods in the social sciences (2nd ed.) (pp. 434-444). New York: Routledge.
Sweet, T. M. (in press). Modeling social networks as mediators: A mixed membership stochastic blockmodel for mediation. Journal of Educational and Behavioral Statistics.
Yang, J. S., Morell, M., & Liu, Y. (2018). Constructed response items. In B. Frey (Ed.), The SAGE Encyclopedia of Educational Research, Measurement, & Evaluation. New York, NY: Sage.
Zhan, P., & Jiao, H. (2018). Using JAGS for Bayesian cognitive diagnosis modeling: A tutorial. Journal of Educational and Behavioral Statistics.
Zhan, P., Jiao, H., Liao, D. & Li, F. (in press). A longitudinal higher-order diagnostic classification model. Journal of Educational and Behavioral Statistics.
Zhan, P., Wang, W.-C., Jiao, H., & Bian, Y. (2018). The probabilistic-inputs, noisy conjunctive models for cognitive diagnosis. Frontiers in Psychology.
Zhan, P., Jiao, H., Liao, M., & Bian, Y. (2018). Bayesian DINA modeling incorporating within-item characteristics dependency. Applied Psychological Measurement.
Zhang, S., Chen, Y., & Liu, Y. (2018). An improved stochastic EM algorithm for large‐scale full‐information item factor analysis. British Journal of Mathematical and Statistical Psychology.
Zheng, X., & Yang, J. S. (2018). Latent growth curve analysis with categorical data: Model specification, estimation, and panel attrition. Multivariate Behavioral Research, 53, 134-135.
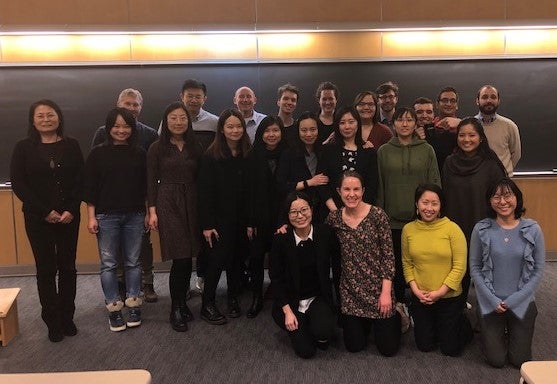
EDMS Research Day 2019