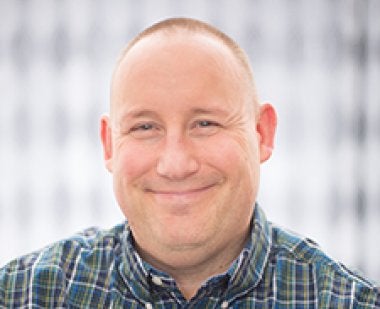
How do you introduce yourself professionally at a dinner party full of non-academics?
Some of my College of Education colleagues say proudly, “Oh, I’m a reading research scientist,” or “I’m a professor of special education,” or “I’m an assistant professor of counseling psychology.” In each of these cases, curiosity and some basic understanding of what these titles mean typically moves the conversation along.
With chest out, I too have answered this inquiry many times, usually with “I’m a statistician,” which is immediately answered with a blank stare, a narrowing of the eyebrows, and an uncomfortable silence. I’d swear I could hear crickets singing in the background. Frequently, it’s enough of a conversation stopper that the unwitting questioner looks for any reason to escape: “My goodness, look at the time,” “Dear, our wine glasses are empty,” or “Did Marge just put out the buffalo chicken dip?”
Brave souls who find the gumption to say something in response often recall a bad memory of a statistics course they took back when pencil sharpeners were bolted to the classroom wall. On occasion, I’ve used the less intimidating but apparently no more illuminating “I’m a quantitative methodologist,” which elicits the same blank stares, minus the angst.
Of course, this odd behavior from non-academics is understandable and, perhaps to some degree, predictable. But I also get these same distant gazes from some of my colleagues in the many programs and departments within the halls of Benjamin. I suppose they might be wondering just who quantitative methodologists are and what we do.
Who We Are
While it’s true that some of my colleagues in this broad, “mathy” discipline can be socially awkward and a bit standoffish, I’m here to report that these traits don’t typify the nice folks in the Measurement, Statistics, and Evaluation (EDMS) program. In fact, we’re a friendly, inviting bunch, brimming with good humor. Yes, we are a bit nerdy, but I’ve never met a quantitative methodologist who wasn’t.
What makes someone want to go into this field? At a basic level, I think people like me end up in this area of research because they find out they’ve got a knack for it. Because the quantitative requirements are not inconsequential, often there has been a pivotal person in our training who was highly influential – someone who was a good teacher or who posed the questions or the approaches in a way that piqued our curiosity. While this can be said about researchers in many domains, it is especially true in quantitative methodology, where it takes some time to attain enough background even to begin making a contribution to the research literature.
As my advisor once suggested, quantitative methodologists are a “type.” I think the rigor of the whole analytic enterprise – this way of getting information and representing the world – appeals to a certain type of person. I particularly enjoy grappling with the computer and the synthesis that occurs when working with “real data,” employing a statistical model like a strainer to help filter what the data present, so that aspects that wouldn’t have come to light without this sort of summarizing become more obvious.
It’s kind of an “art appreciation” issue. The work appeals to us because it is a way to bring order to and to help blend all the disparate sources of information one works with.
What We Do
Many of my colleagues at the College of Education have come to realize that the well-trained, articulate statisticians in EDMS are just the people to bring onto an advisee’s dissertation committee – knowledgeable guides who can often answer questions about the research design or the data analytic plan. At other times, EDMS faculty provide timely advice on data analysis for projects that use sophisticated modeling techniques or approaches. We are also called upon to provide power/sample size calculations – those sinister computations that every grant writer knows are crucial to a successful application. And we spend some of our time answering emails, often with the seemingly benign subject line “A quick question,” from well-meaning colleagues and students who want clarification on a sticky methods issue. For an experienced quantitative methodologist, these questions are usually anything but “quick.”
Yes, these are the extracurricular activities of community-minded quantitative methodologists, yet they are not typically part of the research landscape in our discipline. So what type of research does a quantitative methodologist conduct?
Part of our work is inventing new statistical and psychometric methods. These research activities are usually grounded in a real data problem for which no methodological solution exists. My colleague Dr. Laura Stapleton likens what we do to the work of engineers.
An engineer who develops and tests microwave ovens might be motivated by such questions as: How high can the heat be without melting plastic? What materials can be used in a microwave? Under what conditions will the internal mechanisms break down?
Our products, however, are not physical objects. We’re engineers who develop and test psychometric and statistical models. When you push the start button on a microwave, you might think of the engineering work that was instrumental in making it run. So, when pushing the run radio button in your favorite statistical software program, stop and think about the quantitative methodologists and statisticians who developed that product.
Most quantitative methodologists I know are not pure theoretical statisticians or probabilists or arithmeticians. To some extent, our work is motivated by nuances of research protocols, data collection methods, sampling designs, and of course the data themselves, which are frequently fallible measures of the myriad facets of human behavior. Our charge is to find new methods to analyze data when none exist, as well as to test the veracity of existing methods under suboptimal conditions. Questions like “When would this statistical method break down in practice?” can be central to a methodological investigation.
As a concrete example of just how innovative this work can be, consider a recent book chapter in which my colleagues and I proposed a modeling extension to an existing multilevel modeling framework. We were seeking to accommodate the simultaneous estimation of students’ growth over time when that growth is nested within teachers who are also being assessed longitudinally (Harring, Beretvas, & Israni, 2015). This is methodologically nuanced: students and teachers do not have to be measured at the same time, and students move across different teachers as they progress through school. So, for instance, a researcher might ask, “How do you link students’ growth characteristics to teachers’ growth characteristics for data like these, which are not only nested but have a cross-classified, multiple membership structure?”
Existing statistical methods are incapable of handling this unique data structure in a straightforward manner. The methods we have must be extended in a distinctive way to accommodate data of this type, which incidentally were collected as part of an IES grant examining the effectiveness of a new curriculum in D.C. charter schools. The complexity of the longitudinal data structure necessitated that we create a new model, figure out how to estimate model parameters, and then document it all in such a way that others could utilize the model in the future.
Quantitative methodologists are a funny bunch. When called upon, we strive to illuminate data analytic activities that are integral to many educational researchers’ daily routines. But our primary research goals – the work we were hired to do – involve the creation, development, and refinement of statistical and psychometric methods for answering interesting, substantive scientific questions.
Maybe with this explanation, I can compete with the buffalo chicken dip.
Dr. Jeffrey Harring is an associate professor in the Measurement, Statistics, and Evaluation program in the Department of Human Development and Quantitative Methodology. His research focuses on applications of statistical models for repeated measures data, nonlinear structural equation models, and statistical computing.
References
Harring, J. R., Beretvas, S. N., & Israni, A. (2015). A model for cross-classified nested repeated measures data. In J. R. Harring, L. M. Stapleton, & S. N. Beretvas (Eds.), Advances in multilevel modeling for educational research: Addressing practical issues found in real-world applications (pp. 229-260). Charlotte, NC: Information Age Publishing, Inc.