Jeffrey R. Harring
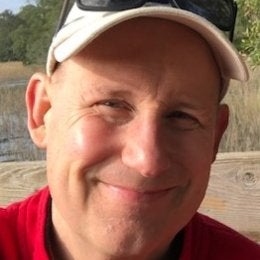
Dr. Harring is a Professor in the Quantitative Methodology: Measurement and Statistics (QMMS) in the Department of Human Development and Quantitative Methodology at the University of Maryland. Prior to joining the the QMMS faculty in the fall of 2006, Dr. Harring received a M.S. degree in Statistics in 2004, and completed his Ph.D. in the Quantitative Methods Program within Educational Psychology in 2005--both degrees coming from the University of Minnesota. Before that, Dr. Harring taught high school mathematics for 12 years.
College of Education Excellence in Scholarship and Research (Tenured Faculty) (2023). University of Maryland.
Society of Multivariate Experimental Psychology. Elected Member (2017).
Recognized at the 9th Annual University-Wide Celebration of Scholarship and Research (2016). University of Maryland.
College of Education & Human Development (CEHD) Rising Alumni Award (2016). University of Minnesota.
University of Maryland Graduate Faculty Mentor of the Year Award (2015). University of Maryland.
College of Education Excellence in Graduate Mentoring Award (2015). University of Maryland.
College of Education Excellence in Teaching Award (2010). University of Maryland.
University of Maryland Graduate Student Mentor of the Year Award Nominee (2013-2014). University of Maryland.
Hancock, G. R., Harring, J. R., & Macready, G. B. (Eds.) (2019). Advances in latent class analysis: A festschrift for Chan Dayton. Charlotte, NC: Information Age Publishing, Inc.
Harring, J. R., & Stapleton, L. M., & Beretvas, S. N. (Eds.) (2015). Advances in multilevel modeling for educational research: Addressing practical issues found in real-world applications. Charlotte, NC: Information Age Publishing, Inc.
Harring, J. R., & Hancock, G. R. (Eds.) (2012). Advances in longitudinal methods in the social and behavioral sciences. Charlotte, NC: Information Age Publishing, Inc.
Zieffler, A. S., Harring, J. R., & Long, J. D. (2011). Comparing groups: Randomization and bootstrap methods using R. New York: Wiley.
Harring, J. R., & Zou, J. (2023). Nonlinear structural equation models: Advanced methods and applications. In R. H. Hoyle (Ed.), Handbook of structural equation modeling (2nd ed.) (pp. 681-700). The Guilford Press.
Harring, J. R., & Blozis, S. A. (2022). Modeling nonlinear longitudinal change with mixed effects models. In A. A. O’Connell & D. B. McCoach (Eds.), Multilevel modeling with introductory and advanced applications (pp. 355-388). Information Age Publishing, Inc.
Liu, J., & Harring, J. R. (2019). A systematic investigation of within-subject and between-subject covariance structures in growth mixture models. In G. R. Hancock, J. R. Harring & G. B. Macready (Eds.). A festschrift for Chan Dayton. Information Age Publishing, Inc.
Harring, J. R., & Houser, A. (2016). Longitudinal models for continuous repeated measures data. In A. A. Rupp, & J. Leighton (Eds.), Handbook of cognition and assessment: Frameworks, methodologies, and applications (pp. 267-296). New York: Wiley.
Harring, J. R., Beretvas, S. N., & Israni, A. (2015). A model for cross-classified nested repeated measures data. In J. R. Harring, L. M. Stapleton, & S. N. Beretvas (Eds.), Advances in multilevel modeling for educational research: Addressing practical issues found in real-world applications (pp. 217-246). Charlotte, NC: Information Age Publishing, Inc.
Stapleton, L. M., Harring, J. R., & Lee, D. (2015). Sampling weight considerations for multilevel modeling of panel data. In J. R. Harring, L. M. Stapleton, & S. N. Beretvas (Eds.), Advances in multilevel modeling for educational research: Addressing practical issues found in real-world applications (pp. 59-91). Charlotte, NC: Information Age Publishing, Inc.
Hancock, G. R., Harring, J. R., & Lawrence, F. R. (2013). Using latent growth models to evaluate longitudinal change. In G. R. Hancock & R. O. Mueller (Eds.), Structural equation modeling: A second course (2nd ed.) (pp. 309-341). Greenwood, CT: Information Age Publishing, Inc.
Harring, J. R. (2012). Finite mixtures of nonlinear mixed effects models. In J. R. Harring & G. R. Hancock (Eds.), Advances in longitudinal methods in the social and behavioral sciences (pp. 159-192). Charlotte, NC: Information Age Publishing, Inc.
Harring, J. R., & Johnson, T. L. (2020). Longitudinal data analysis (ITEMS Digital Module 16). Educational Measurement: Issues and Practice, 39, 137–138. It has been published in final form at https://onlinelibrary.wiley.com/journal/17453992.
Man, K., & Harring, J. R. (in press). Detecting pre-knowledge cheating via innovative measures: A mixture hierarchical model for jointly modeling item responses, response times, and visual fixation counts. Educational and Psychological Measurement.
Dardick, W. A., & Harring, J. R. (in press). A novel numerical method for solving unknown statistical quantities in multivariate regression models. Journal of Educational and Behavioral Statistics.
Harring, J. R., & Harring, E. K. (2023). Estimation approaches: Least squares, maximum likelihood, and Bayesian. In R. J. Tierney, F. Rizvi, & K. Erkican (Eds.), International encyclopedia of education (4th ed.), vol. 14. Elsevier.
Lee, D. Y., & Harring, J. R. (2023). Handling missing data in growth mixture models. Journal of Educational and Behavioral Statistics, 48, 320-348.
Leite, W., Shen, W., Marcoulides, K., Fisk, C., & Harring, J. R. (2022). Using ant colony optimization for sensitivity analysis in structural equation modeling. Structural Equation Modeling: A Multidisciplinary Journal, 29, 47-56.
Fisk, C., Harring, J. R., Shen, Z., Leite, W., King, Y.-S., & Marcoulides, K. (2022). Using simulated annealing to investigate sensitivity to external model misspecification in SEM. Educational and Psychological Measurement, 83, 73-91.
Man, K., Harring, J. R., & Zhan, P. (2022). Bridging models of biometric and psychometric assessment: A three-way joint modeling approach of item responses, response times and gaze fixation counts. Applied Psychological Measurement, 46, 361-381.
McNeish, D. M., & Harring, J. R. (2021). Improving convergence in growth mixture models without covariance structure constraints. Statistical Methods in Medical Research, 30, 994-1012.
Blozis, S. A., & Harring, J. R. (2021). Fitting nonlinear mixed-effects models with alternative residual covariance structures. Sociological Research & Methods, 50, 531-566.
Zheng, X., Yang, J. S., & Harring, J. R. (2021). Latent growth curve analysis with item response data: A methodological investigation of model parameterization, estimation, and attrition. Structural Equation Modeling: A Multidisciplinary Journal, 29, 182-206.
Man, K., & Harring, J. R. (2020). Assessing pre-knowledge cheating via innovative measures: A multiple-group analysis of jointly modeling item responses, response times, and visual fixation counts. Educational and Psychological Measurement, 81, 441-465.
Cassiday, K., Cho, Y., & Harring, J. R. (2020). A comparison of label switching algorithms for finite mixture models. Educational and Psychological Measurement, 81, 668-697.
McNeish, D. M., & Harring, J. R. (2020). Covariance pattern mixture models: Eliminating random effects to improve convergence and performance. Behavior Research Methods, 52, 947-979.
Harring, J. R., Strazzeri, M., & Blozis, S. A. (2020). Piecewise latent growth models: Beyond modeling linear-linear processes. Behavior Research Methods, 53, 593-608.
Feng, Y., Hancock, G. R., & Harring, J. R. (2019). Latent growth models with floors, ceilings, and random knots. Multivariate Behavioral Research, 54, 751-770.
Prendez, J., & Harring, J. R. (2019). Measuring parameter uncertainty by identifying fungible estimates in SEM. Structural Equation Modeling: A Multidisciplinary Journal, 26, 893-904.
Man, K., & Harring, J. R. (2019). Negative binomial models for visual fixation counts on test items. Educational and Psychological Measurement, 79, 617-635.
Man, K., Harring, J. R., & Sinharay, S. (2019). Use of data mining methods to detect test fraud. Journal of Educational Measurement, 56, 251-279.
Man, K., Harring. J. R., Ouyang, U., & Thomas, S. L. (2018). Response time based nonparametric Kullback-Leibler divergence measure for detecting aberrant test-taking behavior. International Journal of Testing, 18, 155-177.
Amaya, A., & Harring, J. R. (2017). Assessing the effect of social integration on unit nonresponse in household surveys. Journal of Survey Statistics and Methodology, 5, 480-508.
Harring, J. R., McNeish, D., & Hancock, G. R. (2017). Using phantom variables in structural equation modeling to assess model sensitivity to external misspecification. Psychological Methods, 22, 616-631.
McNeish, D. M. & Harring, J. R. (2017). Clustered data with small sample sizes: Comparing the performance of model-based and design-based approaches. Communications in Statistics - Simulation and Computation, 46, 855-869.
McNeish, D., & Harring, J. R. (2017). The effect of model misspecification on growth mixture model class enumeration. Journal of Classification, 34, 223-248.
Harring, J. R., & Blozis, S. A. (2016). A note on recurring misconceptions when fitting nonlinear mixed models. Multivariate Behavioral Research, 51, 805-817.
Blozis, S. A., & Harring, J. R. (2016). On the estimation of nonlinear mixed-effects models and latent curve models for longitudinal data. Structural Equation Modeling: A Multidisciplinary Journal, 23, 904-920.
Harring, J. R., & Hodis, F. A. (2016). Applications of mixture modeling in educational psychology. Educational Psychologist, 51, 354-367.
McNeish, D., & Harring, J. R. (2016). Correcting model fit criteria for small sample latent growth models with incomplete data. Educational and Psychological Measurement. DOI: 10.1177/0013164416661824.
Kohli, N., Harring, J. R., & Zopluoglu, C. (2016). Estimation of the finite mixture of nonlinear random coefficient models. Psychometrika, 81, 851-880.
Silverman, R. D., Proctor, C. P, Harring, J. R., Johnson, E. M., Taylor, K. S., Lee, Y., & Jones, R. (2021). The effect of a language and literacy intervention on upper elementary bilingual students’ argument writing. The Elementary School Journal, 122, 208-232.
Panlilio, C., Harring, J. R., & Jones Harden, B., Morrison, C. I., & Duncan, A. D. (2020). Heterogeneity in the dynamic arousal and modulation of fear in young foster children. Children and Health Services Review, 116, 1-29.
Rieger, S., Göllner, R., Spengler, M., Trautwein, U., Nagengast, B., Harring, J. R., & Roberts, B. W. (2018). The effects of getting a new teacher on the consistency of personality. Journal of Personality, 87, 485-500.
Panlilio, C., Jones Harden, B., & Harring, J. R. (2017). School readiness of maltreated preschoolers and later school achievement: The role of emotion regulation, language, and context. Child Abuse & Neglect, 75, 82-91.
Leech, K., Wei, R., Harring, J. R., & Rowe, M. L. (2018). A brief parent-focused intervention to improve preschoolers’ conversational skills and school readiness. Developmental Psychology, 54, 15-28.
Park, J., & Moser, D. K., Griffith, K., Harring, J. R., & Johantgen, M. (2017). Exploring symptom clusters in people with heart failure. Clinical Nursing Research, 28, 165-181.
Proctor, C. P., Harring, J. R., & Silverman, R. D. (2017). Linguistic interdependence between Spanish language and English language and reading: A longitudinal exploration from second through fifth grade. Bilingual Research Journal, 40, 372-391.
Leite, W. L., Shen, Z., Fisk, C. L., Wright, E., Harring, J. R., & Marcoulides, K. (2023, June). A tutorial on methods for sensitivity analysis to omitted confounders in structural equation modeling. Presented at the Modern Methods Modeling Conference, University of Connecticut, Storrs, CT.
Wang, W., Liu, Y., & Harring, J. R. (2022, April). Statistical inference for regularized differential item functioning. Paper presented at NCME Annual Meeting, San Diego, CA (online meeting).
Man, K., & Harring, J. R. (2022, April). Using mixture models to identify aberrant test takers in large scale assessments. Paper presented at NCME Annual Meeting, San Diego, CA (online meeting).
Harring, J. R. (2021, April). A multilevel structured latent curve model for disaggregating student and school contributions to learning. Invited keynote address: Multilevel Modeling Special Interest Group presented at AERA Annual Meeting, Orlando, FL (online meeting).
Fisk, C. L., & Harring, J. R. (2021, April). On conducting a sensitivity analysis in SEM using simulated annealing. In W. Leite (Chair), Sensitivity Analysis in Structural Equation Modeling [symposium]. Paper presented at AERA Annual Meeting, Orlando, FL (online meeting).
Man, K., Harring, J. R., & Choi, Y.-J. (2021, June). Detecting pre-knowledge cheating via innovative measures: a joint structural mixture modeling of item responses, response times, and visual fixation counts. In. J. Wollack (Chair), Innovations in Detection of Test Collusion [Paper Session/Symposium]. NCME Annual Meeting, Baltimore, MD (online meeting).
Harring, J. R. (2019, September). Can residuals be used to understand covariance structures in longitudinal mixture models? Presented at the Innovations of Latent Variable and Random Effects Models Conference, Colorado Springs, CO.
Prendez, J., & Harring, J. R. (2018, May). Exploring fungible parameter estimates in SEM. Presented at the Modern Modeling Methods Conference, University of Connecticut, Storrs, CT.
Cassiday, K., & Harring, J. R. (2018, April). A comparison of label switching algorithms. Paper presented at the American Educational Research Association Conference, New York, NY.
Man, K., Harring, J. R., Sinharay, S., & Jiao, H. (2018, April). Investigating the use of data mining methods in the detection of aberrant testing behavior. Paper presented at the National Council of Measurement in Education Conference, New York, NY.
Zou, J., Harring, J. R., Brandt, H., & Kelava, A. (2018, April). A multilevel IRT model with nonlinear effects. Paper presented at the National Council on Measurement in Education Conference, New York, NY.
Harring, J. R., Zou, J., Brandt, H., & Kelava, A. (2017, April). Nonlinear structural equation mixture models in a multilevel framework: An empirical study. Paper presented at the American Educational Research Association Conference, San Antonio, TX.
Lee, D. Y., Stapleton, L. M., & Harring, J. R. (2017, April). Respondent attrition in longitudinal analysis of panel data. Paper presented at the American Educational Research Association Conference, San Antonio, TX.
Harring, J. R. (2016, August). Exploring model-based covariance structures in finite mixture models. In S. K. Sterba (Chair), Advances in mixture modeling methods. Invited symposium at American Psychological Association conference. Denver, CO.
Man, K., & Harring, J. R. (2016, May). Aberrant behavior detection based on a multivariate mixture modeling approach. Presented at the Modern Modeling Methods Conference, University of Connecticut, Storrs, CT.
Calico, T., Rupp, A. A., & Harring, J. R. (2016, April). Aligning process, product and survey data: Bayes nets for a simulation-based assessment. Paper presented at the National Council of Measurement in Education Conference, Washington, D.C.
Harring, J. R., & Liu, J. (2015, May). Simulating data for mixture model studies: Considering measures of data overlap. Presented at the Modern Modeling Methods Conference, University of Connecticut, Storrs, CT.
Kang, Y., & Harring, J. R. (2012, May). Investigating the Impact of Non-Normality, Effect Size and Sample Size on Two-Group Comparison Procedures: An Empirical Study. Presented at the annual meeting of the American Educational Research Association (AERA), SIG: Educational Statisticians, Vancouver, BC. (Student Paper Award Winner)
Hancock, G. H., & Harring, J. R. (2011, May). Using Phantom Variables in Structural Equation Modeling to Assess Model Sensitivity to External Misspecification. Presented at the Modern Modeling Methods Conference, University of Connecticut.
Kohli, N., & Harring, J. R. (2011, April). Modeling growth in latent variables using a piecewise function. Presented at the annual meeting of the American Educational Research Association (AERA), SIG: Educational Statisticians, New Orleans, LA. (Student Paper Award Winner)
Institutes of Educational Sciences (Goal 3). Evaluating the Efficacy of the CLAVES Intervention: An Intervention Focused on Comprehension, Language Awareness, and Vocabulary for EL Students. $3,200,000
Institutes of Educational Sciences (Goal 3). Toggle Talk. $3,247,480
National Institute of Mental Health. Trajectories of Behavioral Inhibition and Risk for Anxiety. $3,551,633
Institute of Educational Sciences (Goal 2); NCER for English Learners. The CLAVES Intervention Project: Developing a Supplemental Intervention for Comprehension, Linguistic Awareness, and Vocabulary in English for Spanish Speakers. $1,500,000
Institute of Educational Sciences (Goal 1); NCER for Reading and Writing. Investigating Vocabulary Breadth and Depth and Comprehension in English Monolingual and Spanish-English Bilingual Students in Grades 2-5. $1,500,000
Dr. Harring teaches quantitative methods courses making up the QMMS graduate-level statistics sequence: QMMS 646 - General Linear Model I, and QMMS 651 - General Linear Model II. Corresponding more to his own research interests, Dr. Harring has also taught advanced graduate seminars on longitudinal data analysis (QMMS 769L), statistical computing and Monte Carlo simulation (QMMS 779), and finite mixture models in measurement and statistics (QMMS 769M).